Calibrating Marketing Mix Models with Experiments and Attribution data
Model calibration is a new approach in Next Generation Marketing Mix Models (MMM) that can dramatically increase the reliability of MMM results and the accuracy of ROI estimates. For marketing professionals, it means that they can be more confident about using the MMM results in major budget allocation and optimization decisions.
In this article, we will explore why model calibration is so important and how it helps companies make better decisions and spend their marketing budgets more wisely. Our approach to model calibration has been influenced and inspired by Meta’s pioneering work on the topic. Meta’s Igor Skokan has been one of the leading thinkers on calibrating MMMs with experiments, see for example A New Gold Standard for Digital Ad Measurement? (Harvard Business Review 2023). This introductory blog post builds on these ideas, and expands the concept of calibrating MMMs with experiments further to calibrating MMMs with attribution data (ad platform attribution, Google Analytics 4, multi-touch attribution).
Why are Next Generation MMMs using Model Calibration?
Before diving deeper into the technical side of model calibration, we first need to establish that the idea of leveraging model calibration is a major change in the underlying philosophy of estimating marketing’s performance.
The traditional MMM philosophy followed by the majority of MMM industry still today has its roots in MMM’s origins in econometrics and statistics. The traditional MMM philosophy can be summarized as “the model has all the answers''. This perspective emphasizes the time-series model as the sole source of truth, with the traditional MMM dataset as an input (raw time-series data for sales and marketing activities). Traditionalists are cautious about incorporating non-traditional data, for example incrementality tests and attribution data, into the model, arguing that this data introduces biases to the model and that the information they bring should already be inherently present in the input data.
Traditionalists’ strong focus on modeling has led to major developments in modeling methods over the years. The MMM industry has advanced from simple linear regression models to more complex Bayesian models, and methods for assessing model validity have improved. Despite these developments, the traditional MMM approach still suffers from fundamental challenges in MMM, such as “low signal-to-noise [ratio]” in the data, as noted in Challenges and Opportunities in Media Mix Modeling (Google Research 2017) and endogeneity especially in online media. When you combine this with a large amount of features and parameters in MMMs, such as coefficients, diminishing return effect parameters and adstock parameters, it means that it’s possible for anyone to build models which can show green on several statistical tests, but still produce ROI estimates that are nonsensical and disconnected from reality.
Tackling this challenge has inspired the emergence of Next Generation MMMs, which we are passionate about at Sellforte. The main thesis of Next Generation MMMs is that the quality of MMM results can be significantly improved by broadening the amount of information given to the model.Next generation MMMs embrace the use of incrementality tests and attribution data which both contain information on marketing’s performance. Because this non-traditional data has certain biases, for example last-click will overestimate ROI of branded search, Next Generation MMMs have techniques to recognize and manage the biases, so that the information they contain can be used to enhance the MMM results. The picture below illustrates how Next Generation Marketing Mix Models leverage both the traditional modeling data, as well as calibration data (incrementality tests and attribution data):
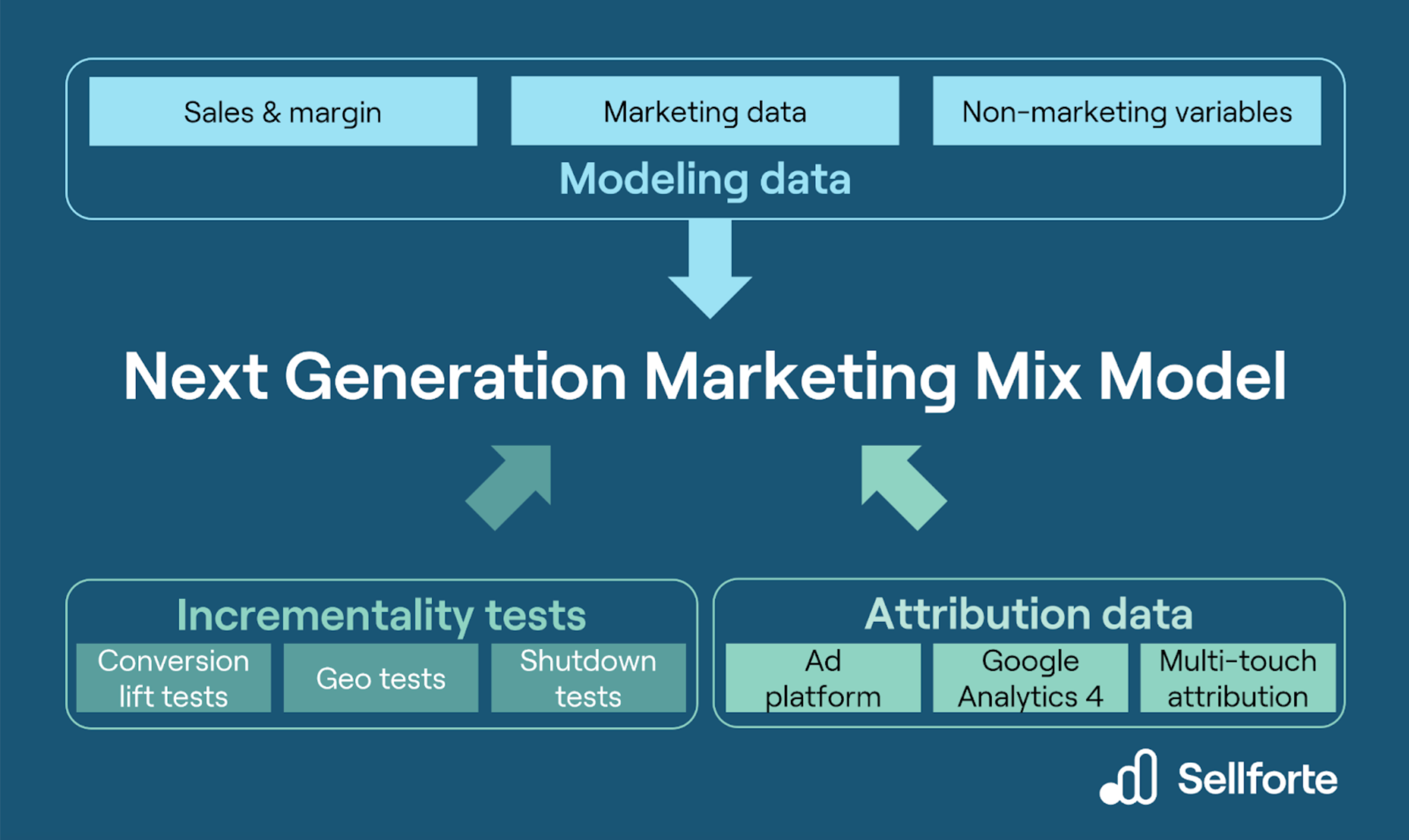
Model calibration is an important part of Sellforte’s vision for Next Generation MMMs. We believe that by combining MMM with incrementality tests and attribution data, our customers will get more accurate & reliable MMM results, as well as a more granular view of their marketing ROI. Instead of looking at experiments, attribution data and MMM as independent or isolated analyses, we combine all the relevant data and analyses together. That is, we solve the problem that marketers face: how to combine different results and what to trust and how much.
How does Model Calibration work?
In the Bayesian modeling approach, the golden standard in MMM today, the model is given "priors" that contain information about the likely range of the ROI of a marketing activity.
Priors are initial beliefs or assumptions about a parameter before any data is observed. For example, if you are predicting the likelihood of rain tomorrow, your prior might be based on the typical weather patterns for that time of year. Priors are formulated as probability distributions, as illustrated below.
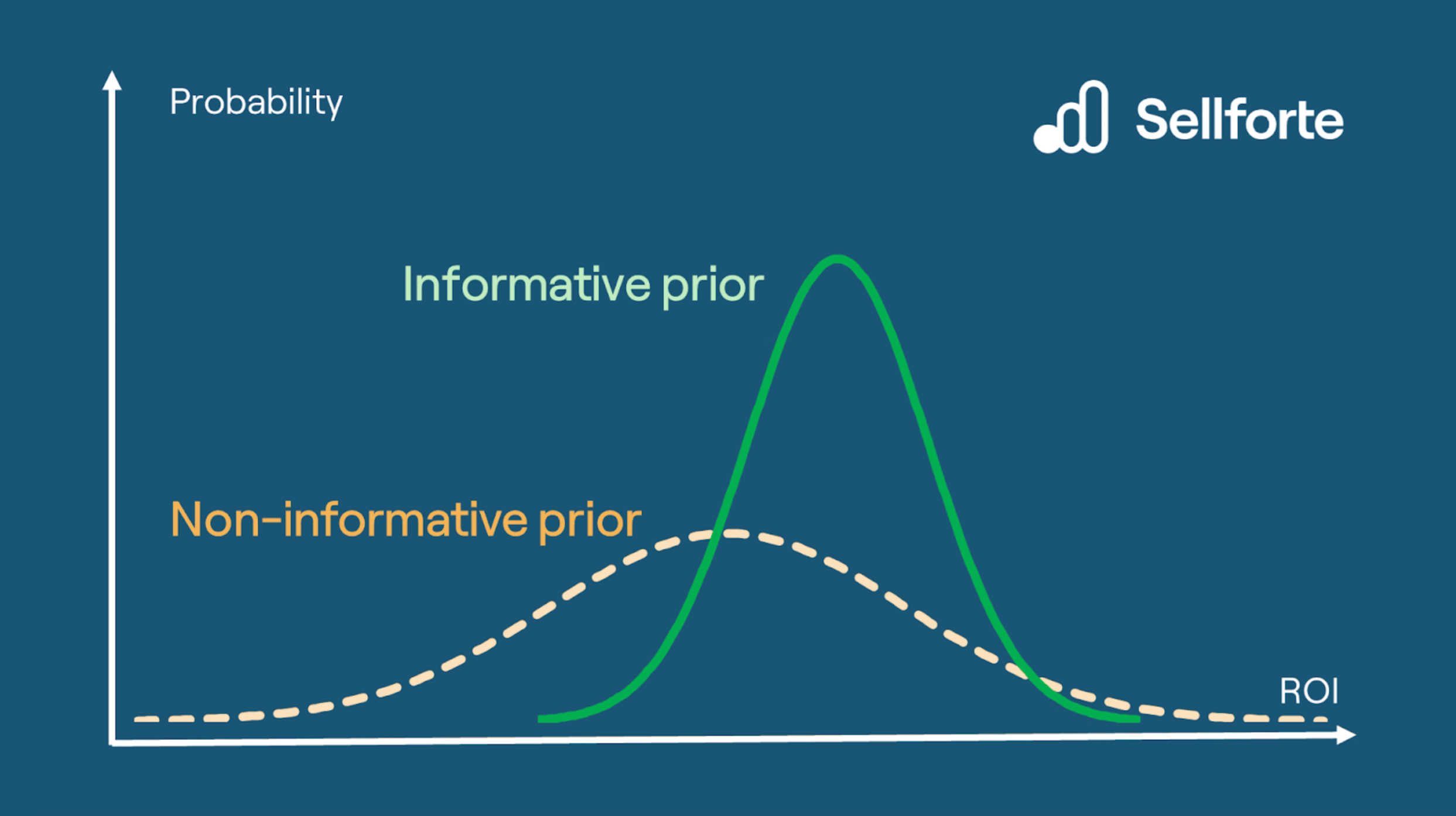
If a non-informative ROI prior is used, there is an attempt to avoid giving the model prior information or assumptions about the effectiveness of a marketing activity. Non-informative prior distributions are wide and flat. The traditional MMM philosophy favors using non-informative priors.
If an informative ROI prior is used, the model is given prior information on the effectiveness of a marketing activity, based on information outside the model. Informative prior distributions are narrower, but the width of the prior distribution can vary depending on the strength of the information that was used to form the prior. Note that despite using informative priors, the model still estimates the final ROI!
By using informative priors, Next Generation MMM combines the power of the model to estimate marketing’s ROI and the richness of the information about marketing’s performance that exist about the ROI outside the model.
What data to use in Model Calibration?
The main assumption in model calibration is that you have strong evidence, which justifies using prior distributions that limit the range of possible ROI estimates. This makes model calibration tricky - you need to be REALLY SURE that you understand the biases and shortcomings in the calibration data that you use. The only way to achieve this, is to have a systematic calibration framework, which guides the calibration process.
We typically leverage two types of data in our calibration framework, when forming prior distributions: Incrementality tests and Attribution data.
1. Incrementality tests in Model Calibration
There’s three main types of incrementality tests that are generally considered in model calibration:
1. Conversion lift tests. The main advantage of conversion lift tests is that their test setup resembles randomized controlled trials (RCTs), which are typically considered one of the best methods for estimating causal effects. RCTs have been widely used in causal experiments across different domains. Availability of conversion lift tests at the time of writing this article is rather limited. Some examples include Meta’s Conversion Lift test and Google’s Conversion Lift test. Additionally, similar experiments could be configured also with A/B tests, such as TikTok’s Split test. The main idea in conversion lift tests is to divide the target audience into test group and control group, and derive insights about marketing’s incrementality by comparing the two groups. Typically, the test group sees the specific ad to be tested, and the control group sees a blank space.
2. Geography tests (Geo tests): Geo tests use a similar concept of creating control and test groups, but here we are talking about geographies. For example, you could divide U.S. states or U.S. Designated Market Areas (DMAs), into test and control areas. The idea is to then apply a treatment to the test group, and compare the groups against each other, to make conclusions about incrementality of a marketing activity. While conversion lift tests typically produce standardized outputs that include estimated value and its confidence intervals, geo tests can be more challenging to analyze, as the method lacks a similar level of standardization. As one example of a Geo test calibration approach, you could check Meta’s GeoLift whitepaper from 2022.
3. Shutdown tests: Shutdown tests involve temporarily stopping a marketing activity, so that the "on" and "off" period can be compared to make conclusions about marketing activity’s incrementality.
2. Attribution data in Model Calibration
In addition to formal tests & experiments, information about marketing’s performance can also be derived from non-experiment data, most notably attribution data. Typical attribution data sources include
- Ad platforms,
- Google Analytics 4, and
- Multi-touch attribution solutions.
While the idea of calibrating MMMs with incrementality tests has already started to gain some traction from the industry, the idea of combining MMM and attribution data, especially in model calibration, is still in its infancy. From the bigger players, we started seeing some developments towards combining MMM and attribution data in Google’s 2024 Modern Measurement Playbook, which discusses the idea of estimating campaign level ROIs by correcting attribution-reported ROAS with incrementality factors estimated by MMM.
The use of attribution data in model calibration is significantly more complex compared to incrementality tests. While incrementality tests might produce one or more estimates for the level of marketing activity’s incrementality under certain conditions, attribution data requires intelligent preprocessing before it reveals information about marketing activity’s likely ROI. For example, it would be a bad idea to use last-click -estimated ROAS directly as the mean of a prior distribution, since last-click attribution as a method contains well-known biases, such as over-reporting ROI of media channels that are close to conversion. Ad platform attribution, on the other hand, typically captures lots of conversions that were not driven by the ad platform, such as conversions driven by other media or conversion that would occur even without marketing. This means that Ad platform -reported ROAS tends to be inflated compared to MMM-reported ROI.
The fact that there’s biases in attribution data does not mean the data cannot be used in model calibration. In fact, our experience is that many of the biases are predictable. When applying the right transformations, attribution data produces lots of information that can be used to triangulate marketing activity’s likely ROI.
Triangulating the likely range of marketing’s ROI with attribution data can be used to complement incrementalitys test results, or used by itself in the absence of tests & experiments. This method can be particularly useful for marketing activities that have low spend, because (i) the benefit of conducting tests described above might not be worth their cost for low spend media, (ii) MMMs are typically better at estimating ROI for larger spend since their impact is more visible on sales.
For more specific methods related to leveraging attribution data in model calibration, we will write a separate blog post.
Criticism against Model Calibration
Using model calibration is a new feature in Next Generation MMMs, so it has received its share of healthy criticism, both from proponents of the traditional MMM philosophy, but also from ourselves at Sellforte as we’ve gone through the process creating our Model Calibration approach. Let’s go through the main points.
1. “So, you are just telling the model what the answer is? You are not really modeling anymore?”
This is a common misconception. The model still estimates the final ROI, based on the time-series data given to the model, but also based on prior information about the ROI. Additionally, the width of the calibration-provided prior distribution can vary depending on the strength of calibration data.
2. “You will misguide the model if you use low quality experiments or other low quality calibration data”
Completely agreed! It is very easy to misuse experiment data or attribution data in model calibration. If you create narrow priors based on faulty evidence, you are forcefully guiding the model to an inaccurate outcome. This is the main reason why we don’t recommend model calibration, or conducting marketing mix modeling yourself in general, unless you know exactly what you’re doing. Model calibration can be done successfully only if you have a calibration framework that includes a process for understanding the data you’re using, and a process for translating that knowledge into meaningful prior distributions.
3. “Incrementality tests are already inherently part of the MMM data and they should be picked by the model. No calibration or incrementality tests needed”
This argument is based on the notion that MMM datasets contain variance both in the sales data and in marketing data, and in a way this variance can be considered as “continuous incrementality testing”. For example, you could naturally have a shutdown test in the data, if you have an “off” period in your media plan, and the model should pick up that information.
The modeling does pick up the signals where they are strong, this is what the model is built for. However, this argument assumes that the signals in MMM datasets are so strong consistently for all media channels and throughout the modeling timeframe, that additional external data, such as additional incrementality tests do not bring any additional value. In practice, this is not the case in our experience, and has not been the experience in the industry more broadly, as noted inthe 2017 Google paper introduced earlier in this blog post.
4. “Rather than using model calibration, you should use econometric approach X, Y, Z in your model”
You should always use the best possible econometric, statistical etc. approaches to have the best possible modeling approach. However, that is not an argument for disregarding the use of marketing performance information contained in incrementality tests and attribution data to generate more accurate and reliable results. It’s not either or, it’s both!
5. “Tests are expensive and laboursome to execute”
This can be true. As a general rule, we recommend spending the most time and money in experimentation and model development in areas where you have the largest opportunity to optimize marketing. This means that it’s typically a good idea to have more regular incrementality testing routine for marketing channels with highest spend, less regular testing for the media next on the list, and potentially only attribution data -based calibration for the media that have very low spends (e.g., 0-5%). A good cost to benefit analysis goes a long way here.
Conclusions
Why should you as a marketer care about model calibration? Three reasons:
- You will get high quality MMM results that are based on harnessing the modeling power of MMMs, but also all available external information on media performance
- Based on the high quality results you will be able to make budgeting decisions confidently
- Making budgeting decisions based on bad quality MMM results is a big risk to your business and to your career
What questions should you ask from your MMM vendor or in-house team to evaluate how they are approaching model calibration?
- What is your model calibration framework? How are prior distributions formed? What data is used to calibrate the model?
- How is the model leveraging incrementality tests?
- How is the model leveraging attribution data from ad platforms, Google Analytics 4, and multi-touch attribution?
Curious to learn more? Book a demo.
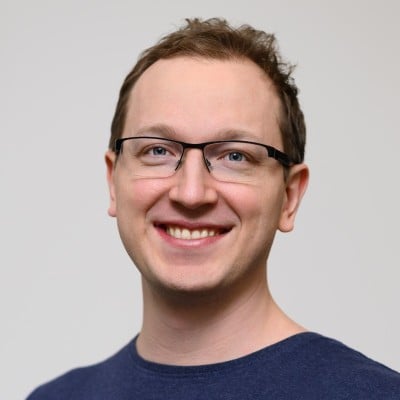
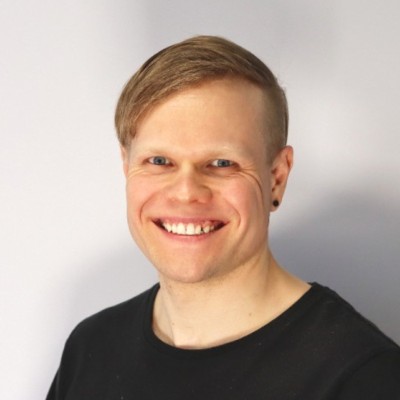
You May Also Like
These Related Stories