Why Marketing Mix Modeling projects fail
Marketing Mix Modelling (MMM) projects have a somewhat bad reputation; traditionally they’ve been cumbersome, grueling, and reactive – just the opposite of what marketing is by nature. Here are some of the most excruciating pitfalls of MMM projects
Whenever a group of marketers gathers around a campfire to share some of their scariest stories, MMM projects are a surefire hit. You feel the tension in the air as the narrator describes the arduous data gathering, see with your mind’s eye how the expectations grow to monstrous proportions, and remain in the dark as the culprits vanish into thin air after the project is finished.
Although the story above is completely made up, there is a hint of truth in it. As the writer and producer J. J. Abrams put it, “Every story has at least a little truth in it. Every story comes from somewhere.”.
Let’s take a look at some of the biggest reasons behind bad MMM project experiences.
Getting the fundamentals of MMM right
MMM projects are usually kicked off when a company seeks to understand how past marketing investments have performed to discover and leverage optimization potential within them.
This is where the company ordering the MMM project needs to be careful, as many providers will promise you the silver bullet for your problems without a second thought about what it takes to solve them.
Making sure all parties are on the same page is one of the most important tasks in MMM projects
As the typical underlying question in MMM is “How much incremental sales/ conversions/demand my marketing investments have driven?”, the modeler needs to first answer “how much sales/conversions/demand there would’ve been without any short-term marketing?”. And this is where you can spot early on if your MMM project is going to fail.
The most prominent red flag here is trying to run the modeling with too little data, as this typically leads to the following issues:
- Not being able to measure the seasonality of demand
- Not being able to measure external variables’ effect on demand (such as Covid-19)
- Not being able to measure the long-term growth of the demand
As MMM is all about the incrementality of marketing (unlike attribution modeling), it is imperative to start with the base. Otherwise, your project is doomed to fail from the start.
Expect the unexpected
The second pitfall lies in setting the expectations for the MMM project. The client and the provider need to be brutally realistic to avoid mismatching the needs and capabilities within the following areas:
- What are the total costs for the project 💸
- What is the projected time-to-results ⏱️
- What insights and actions the project leads to 💡
Mismatched costs
What the modeler charges for the project can vary a lot from what the total cost for the project turns out to be.
You need to fetch the data, clean it, structure it, upload it, define relevant variables and factors, validate the first results… and while some of these costs are included in the initial project costs, there are almost always hidden costs that weren’t considered from the start.
This is also where the build vs. buy comparison has its most prominent differences. Developing an in-house solution can seem quite a desirable option: Like building a house by yourself vs. renting an apartment.
Building a house can seem like a nice idea - until you step on a (data)Lego block
The challenge with in-house MMM projects is that you’re typically building a house for the first time, so you’re not exactly sure what you need or how long it takes. And while tangible costs might sound like the biggest business risk, time is usually much more costly as we’re about to find out…
Mismatched timeline
One of the easiest things to miscalculate when estimating the project roadmap is usually the time-to-results.
Things that can hinder project progress:
- Data quality is not as good as it’s thought to be
- Data is scattered and difficult to fetch
- The initial results are not plausible
Usually, one problem leads to another, creating a domino effect that’ll slow down the process to a standstill. Opening the knots ties in more costs, as somebody has to look into the problem and try to solve it, but missing the deadlines has other implications as well:
- The resources (planned and unplanned) are kept from other initiatives
- Context & business objectives might change (e.g. from cost efficiency to optimizing for growth, or vice versa)
- The current budget allocation remains suboptimal (the cost of inaction)
Considering most MMM projects are launched to discover and leverage optimization potential within the marketing investments, missing the insights while retaining resources from other projects can quickly become the costliest pitfall for MMM.
“Considering most MMM projects are launched to discover and leverage optimization potential within the marketing investments, missing the insights while retaining resources from other projects can quickly become the costliest pitfall for MMM”
Mismatched outcome
As weird as it may sound, the modeling results are the last pitfall that can ruin a MMM project.
“How?” you might wonder.
Providing results is one thing, but delivering credible & actionable results is quite another.
Unfortunately, it’s not uncommon for the client to end up with a MMM project summary that’s either too high-level, unrealistic or both. The client usually knows a lot more about the industry and business, which makes it easy to spot these errors.
Here are a couple of examples of results that are difficult to act upon:
- Too generic results (e.g. ROMI for TV, Meta, Google, Print, OOH…) --> Difficult to interpret which media channels to invest in within the media group
- Unconvincing results:
- Same Media ROMI for all campaign types
- Minimal base sales/demand/brand equity
- Not considering advertised items as a factor
- Static results (not showing/considering Diminishing Return curves) --> No information on what happens to the ROMI on different investment levels
Checklist for MMM project done right
After going through what can go wrong with MMM projects, it’s probably nice to provide some guidelines to avoid these pitfalls with your next MMM project:
- Obsess over incrementality. MMM is all about proving cause and effect, which requires rigorous data analysis
- Challenge the initial costs and project timeline. Being brutally honest with the MMM provider can be hard, but it’s worth it in the end
- Iterate, iterate, iterate. Although we’ve talked about MMM projects in this article, MMM should be approached as a continuously developing process. Marketing is a marathon, so what’s the point in measuring your pulse sporadically
Want to learn more about what kind of options there are for MMM projects? Check out our comparison of different MMM solutions.
Curious to learn more? Book a demo.
You May Also Like
These Related Stories
23 Marketing Mix Modeling Tools for Accelerating Growth in 2025
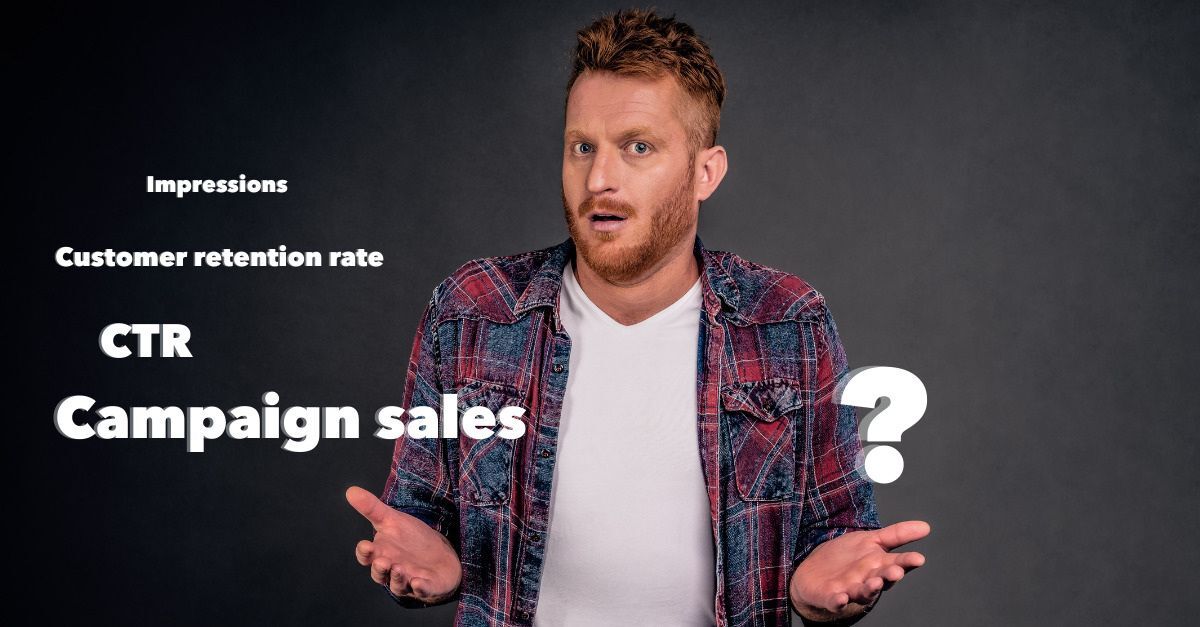