Comparison of Robyn vs Lightweight vs Marketing Mix Modeling SaaS
In today's data-driven world, businesses need to analyze the impact of their marketing efforts to make informed decisions about their strategies. In order to achieve that, they often rely on marketing analytics tools that use data to derive insights. Three popular tools that are available in the market that enable marketers to analyze their marketing efforts are Robyn, Lightweight, and Marketing Mix Modeling presented as software as a service (MMM SaaS). While we understand that MMM SaaS is a whole chapter in itself, we still believe it is relevant to keep it in the background as a comparison element for the “diy” MMM solutions. All three of these options are essentially variations of MM which we will use as a generic term in this article.
Regardless of the fact that each of these tools has its unique features and capabilities, businesses may struggle to choose the right one for their needs. In this blog, we will compare these three marketing analytics tools and discuss their advantages and disadvantages. We will provide an in-depth analysis of their features, pricing, and ease of use to help businesses make an informed decision.
Why even use Marketing Mix Modeling?
Marketers rely on various tools and techniques to measure the effectiveness of their campaigns and make informed decisions about their marketing strategy. One such tool is marketing mix modeling (MMM), which allows marketers to assess the performance of their campaigns and determine how much money to allocate to each media channel.
MMM provides several benefits to marketers. Firstly, it enables comparisons between online and offline media channels which allows marketers to determine the most effective channel for their campaign. Additionally, MMM uses aggregated data to measure marketing effectiveness therefore, it does not require the use of third party cookies, which makes it a reliable and secure way to measure campaign performance.
By using MMM, marketers can calculate the ideal budget distribution among media channels, ensuring that their marketing spend is optimized for maximum impact. They can also examine how each media channel affects their key performance indicators (KPIs), enabling them to make data-driven decisions about which channels to prioritize.
Another advantage of MMM is that it allows marketers to understand how media channels behave when marketing spending changes. By analyzing the impact of different budget allocations on each channel, marketers can adjust their strategy and make more informed decisions about how to allocate their marketing budget.
In-house MMM solutions allow for complete control over the data and modeling processes but will be more expensive due to the need for resources, specialized skills, and time to build them. Purchased solutions may be less customizable, but they offer professional expertise. Also, purchased solutions often provide higher levels of performance and scalability, which is especially beneficial for rapidly growing businesses. However, the decision-making process heavily depends on you and your team.
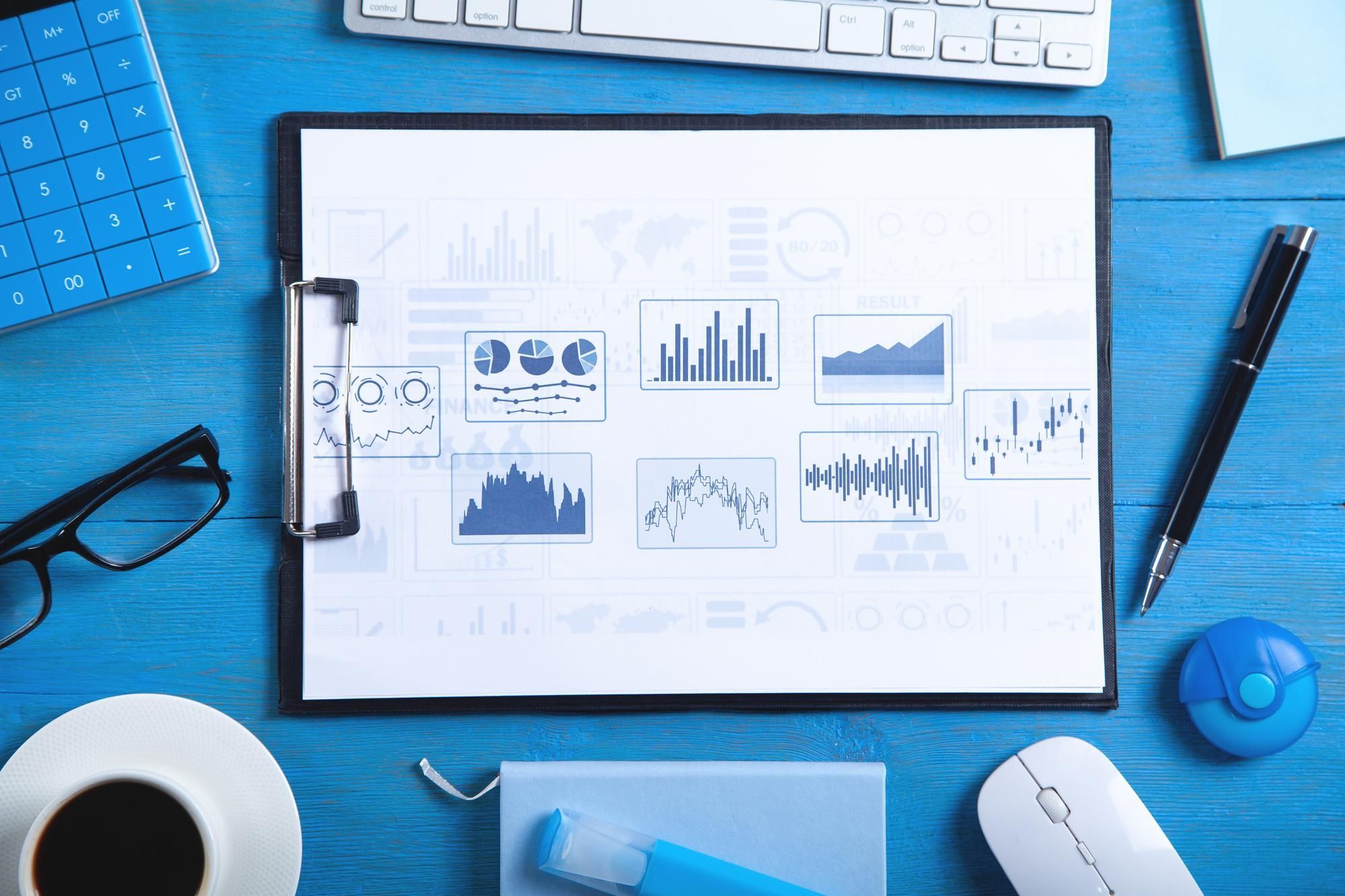
What are Lightweight & Robyn?
The era of cookie-based marketing strategies is rapidly coming to an end. As a response, both Meta & Google launched their own versions of MMM as an open-source library for anyone to use and customize. In this article, we want to show you the similarities and differences between the two in-house MMM options Google's Lightweight MMM (LMMM), and Meta's Robyn MMM (RMMM).
Comparing LMMM and RMMM with MMM SaaS is rather tricky due to the completely opposite nature of the services. Imagine this: you could make your own music in your office all on your own. You could learn to play several instruments, cut the mixtapes, and play them several times until they sound right, or you could buy a music streaming service. Depending on your abilities and goals, one of the two options might be more suitable. Therefore, in full transparency, we think it is best to keep SaaS MMM as its own category.
Lightweight MMM is an open-source Python library that provides marketers with a powerful tool for conducting Bayesian Marketing Mix Modeling. Using the Bayesian algorithm and Numpyro as a backend, the library allows users to integrate their existing data into the model, providing greater accuracy and precision in the analysis.
One of the key advantages of using Bayesian modeling in marketing is that it provides a flexible framework for dealing with uncertainty and variability in the data. By using probability distributions to represent uncertainty, Bayesian models can generate more accurate predictions and insights than traditional linear models. You can read more about Bayesian models and their accuracy here.
Moreover, the fact that Lightweight MMM is an open-source library means that it is freely available for anyone to use, modify and contribute to. This has helped to build a vibrant community of developers who are constantly improving the library and adding new features.
Robyn MMM is also an open-source library, but it uses a different set of tools and technologies than Lightweight MMM. Instead of Numpyro and Python, Robyn MMM uses R and Facebook's Nevergrad Python library, as well as the Prophet library.
R is a popular language for statistical computing and data analysis, and it provides a wide range of tools for linear and nonlinear modeling, calculation, testing, visualization, and analysis. In contrast, Nevergrad is a Python library for optimization that uses modern machine learning techniques, such as Bayesian optimization and genetic algorithms, to find the best parameters for a given model.
In addition, Robyn MMM uses a technique called hyperparameter optimization, which involves running the model thousands of times with different parameters to find the best combination of parameters that produces the most accurate results.
While Python is growing in popularity due to its beginner-friendly readability and open-source availability, R remains a popular choice for statisticians and data analysts due to its powerful tools and features for data analysis and visualization.
Here are some of the key features and benefits that both MMM solutions offer:
- Measure the impact of marketing activities: They allow users to analyze the impact of different marketing activities and channels, such as advertising, promotions, and social media.
- Identify the most effective marketing channels: By analyzing the impact of different marketing activities, both MMM solutions can help businesses identify the channels that are most effective for reaching their target audience and achieving their marketing goals.
- Optimize marketing spend: Both MMM solutions can help businesses optimize their marketing spend by identifying the channels and activities that provide the highest return on investment (ROI).
- Data integration: Both solutions enable users to integrate their own data into the model, allowing for greater accuracy and precision in the analysis.
- Open-source: Both Robyn MMM and Lightweight MMM are open-source libraries, which means that they are freely available for anyone to use, modify and contribute to. This makes them an accessible and cost-effective choice for businesses of all sizes. In comparison, SaaS MMM will most likely be a subscription or fee-based solution.
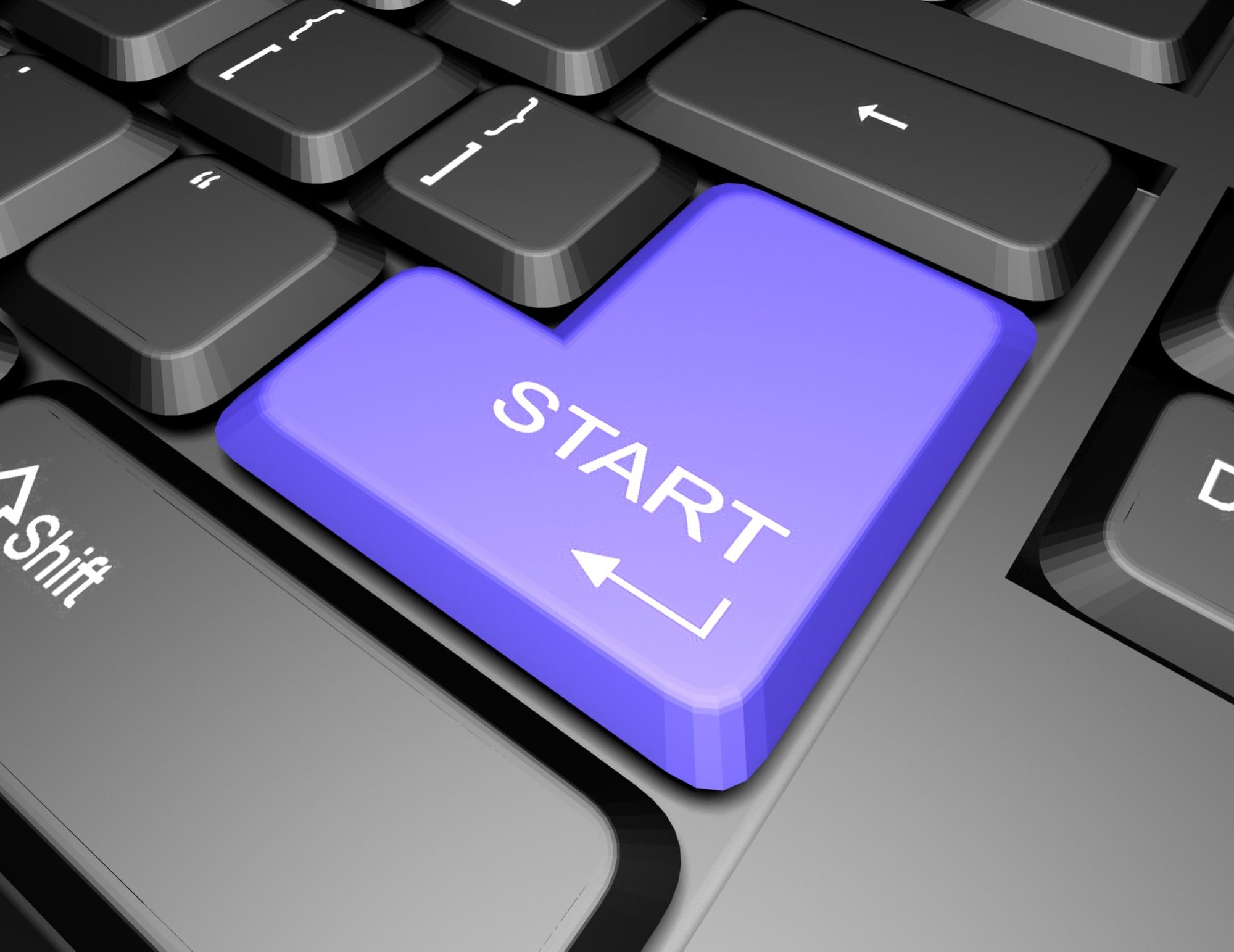
What do you need to start an LMMM, RMMM or SaaS MMM project?
To get the most out of these tools, businesses may need to hire a dedicated data science resource who has expertise in statistical modeling, data analysis, and programming. This resource would be responsible for setting up the models, integrating the data, running the analyses, and interpreting the results.
Moreover, it's important that the data scientist handling the project has a good understanding of marketing concepts and goals. This will help ensure that the model reflects the reality of the business and aligns with its overall objectives. For example, if a business is focused on increasing brand awareness, the data scientist would need to be able to adjust the model accordingly and provide recommendations on the most effective marketing channels and activities to achieve that goal.
In comparison, SaaS does not require any technical knowledge on your side since you receive a finished product. SaaS MMM solutions typically provide a finished product that is easy to use, with little to no technical knowledge required on the user's part. However, you will not have access to the source code, and the product will most likely be subscription based instead of open-source.
Setting up Lightweight MMM or Robyn MMM can be a complex process that requires careful planning and attention to detail. Both solutions have in-depth setup tutorials that guide users through the process, but there are some common requirements and steps that need to be taken.
One of the first steps in setting up either LMMM or RMMM is to organize the data and define the scope of the project. This involves identifying the data sources that will be used in the model, cleaning and preparing the data for analysis, and defining the variables and metrics that will be used to measure the effectiveness of the marketing mix.
Neither Leightweight MMM nor Robyn offers any tools for dealing with the data (getting the data in the first place, validating it, updating it, etc). These tasks typically fall under the domain of data engineering or data management and may require separate tools or expertise to accomplish effectively.
Once the data is organized and the scope of the project is defined, the next steps involve setting up the model and configuring the parameters. This involves selecting the appropriate modeling techniques and algorithms, tuning the hyperparameters, and validating the model against historical data.
In comparison, SaaS also requires an initial onboarding and data review, however, most of the data integration is provided by the vendor. In terms of timeframe, SaaS might take less time due to the experience of the team that implements it; however, the timeframe is heavily dependent on the data condition as well.
What kind of insights do you receive?
Once your model is up and running, your team will have to create plotted outputs from the data so that it is easy to visualize and understand the results. These are highly customizable depending on your scope, however, there are some differences between the LMMM and RMMM outputs.
In terms of output, Lightweight MMM provides estimates of the impact of marketing activities on sales, as well as estimates of the return on investment (ROI) for each activity. It also provides insights into the effectiveness of different marketing channels, such as TV, radio, digital, and print, and allows users to simulate the impact of changes to their marketing mix.
Robyn, however, provides more detailed outputs, including estimates of the impact of marketing activities on different stages of the consumer journey, such as awareness, consideration, and conversion. It also provides insights into the relative importance of different marketing channels, and allows users to simulate the impact of changes to their marketing mix based on different scenarios.
Another key difference between the two solutions is the modeling approach they use. Lightweight MMM uses a Bayesian modeling approach, which is based on probabilities and allows for greater flexibility in modeling complex relationships. In contrast, Robyn MMM, uses a gradient boosting approach, which is a machine learning technique that allows for more complex and non-linear relationships between variables.
Below you have a few potential examples for both MMM solutions:
LMMM natively allows geo data visualization. Below you have an example of what distributions of the media effects, one for each channel-geo combination could look like. The distributions come from the modeling itself, meaning that the reason that we have distributions here is because the model is bayesian. Only because of this factor we can see the uncertainty of the estimate of media effect for each channel.
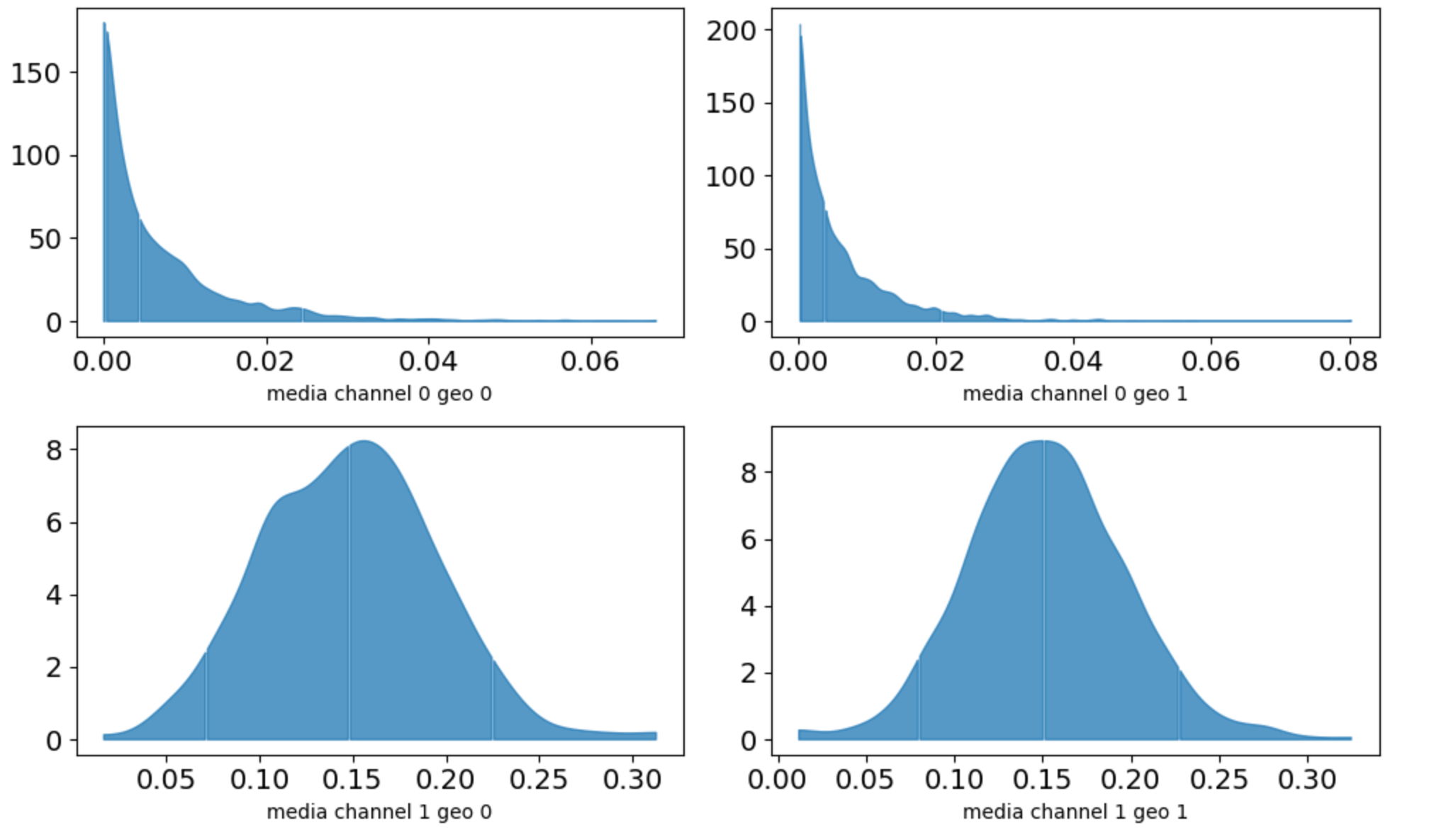
RMMM uses Meta’s Prophet library which allows the user to differentiate amongst trends, seasonality, events and weekdays. Below you have an example of what the plots could look like using the Prophet decomposition function.
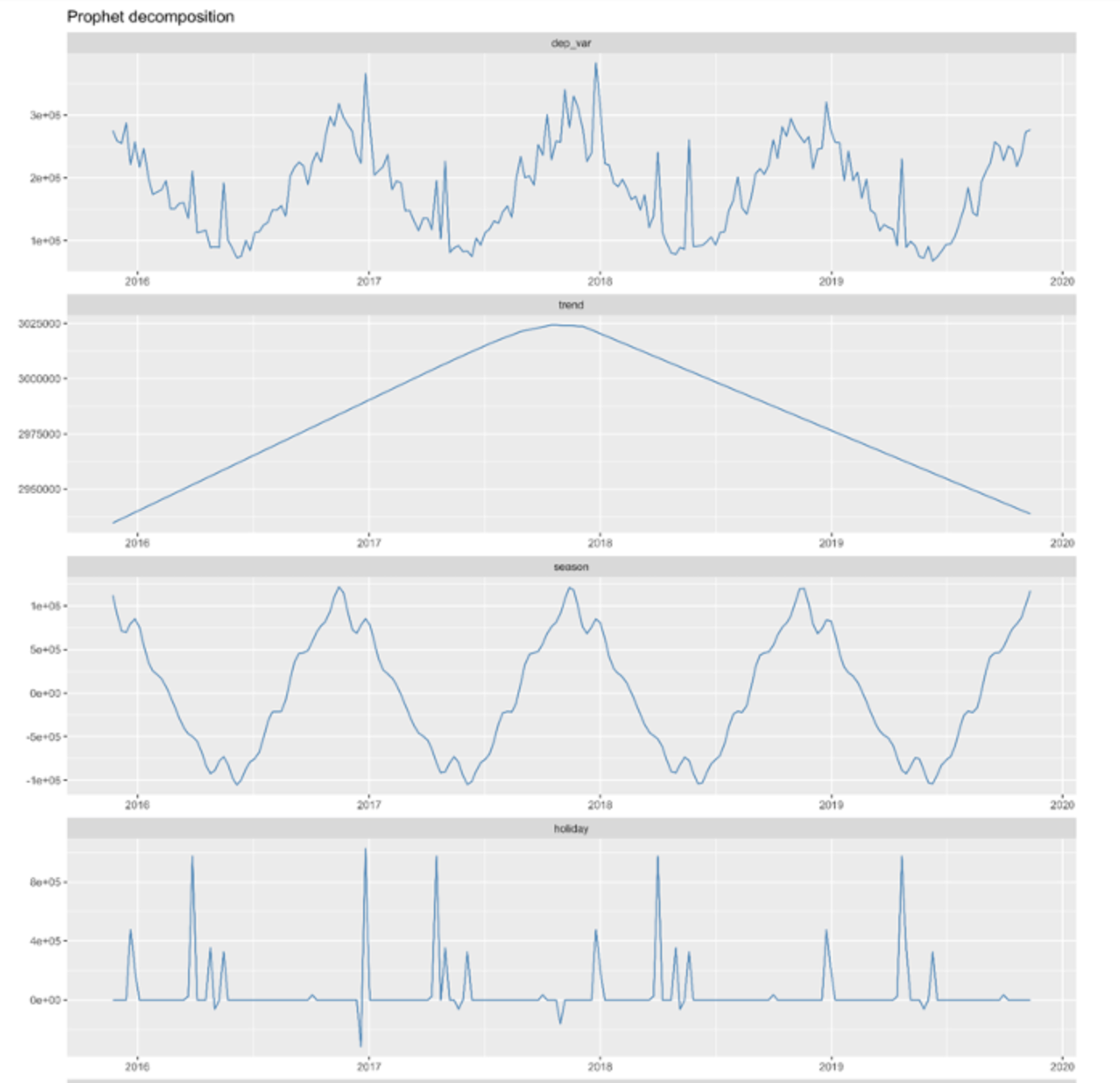
LMMM does not have an interactive user UI. Instead, users would need to build their own UI or run the package using code in a terminal or notebook. On the other hand, Robyn MMM has a web-based interface built using the Shiny framework. This allows users to interact with the model and view the results in a more user-friendly way, without having to write any code.
The availability of a UI can be an important consideration when choosing between marketing mix modeling solutions, because it can make it easier for non-technical users to interact with the model and understand the results.
Lightweight MMM uses Numpyro and JAX for probabilistic programming, which allows it to process data more quickly than Robyn MMM. However, the exact processing time will depend on the amount of data being analyzed and the capabilities of the user's computer. In general, Lightweight MMM is designed to be a faster and more lightweight solution than Robyn MMM.
Robyn MMM can take longer to process data and generate outputs, since it uses R and the Nevergrad Python library for optimization. However, once the modeling process is complete, users are provided with a comprehensive one-pager report. SaaS MMM has a more interactive interface with various dashboards. The results are displayed over several minutes. However, SaaS is also a full time business for professional teams; therefore, it is expected to have a higher level of service in comparison to open source solutions. Hence, in terms of outputs and service, comparing the three is like comparing apples and oranges.
As mentioned before, both LMMM and RMMM solutions offer an optimizer feature that is meant to help allocate media investment. The budget allocation is meant to help you allocate your budget across your media investments in order to maximize ROI. This feature is also standard across all serious SaaS MMM providers.
Below you can see an example of RMMM and LMMM budget allocation output:
Robyn MMM:
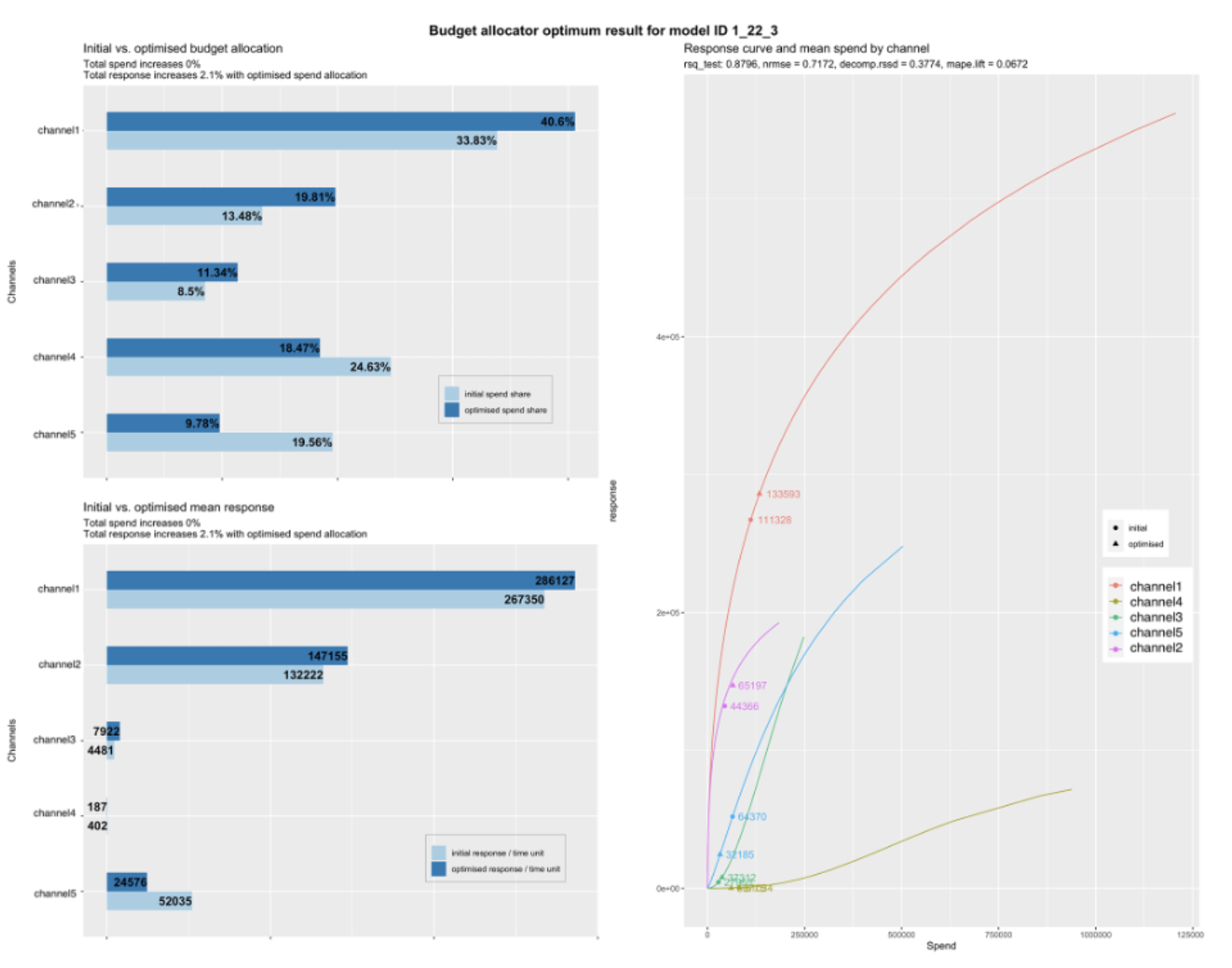
Lightweight:
Unfortunately the results cannot be displayed together as a one page which makes the UI less attractive.
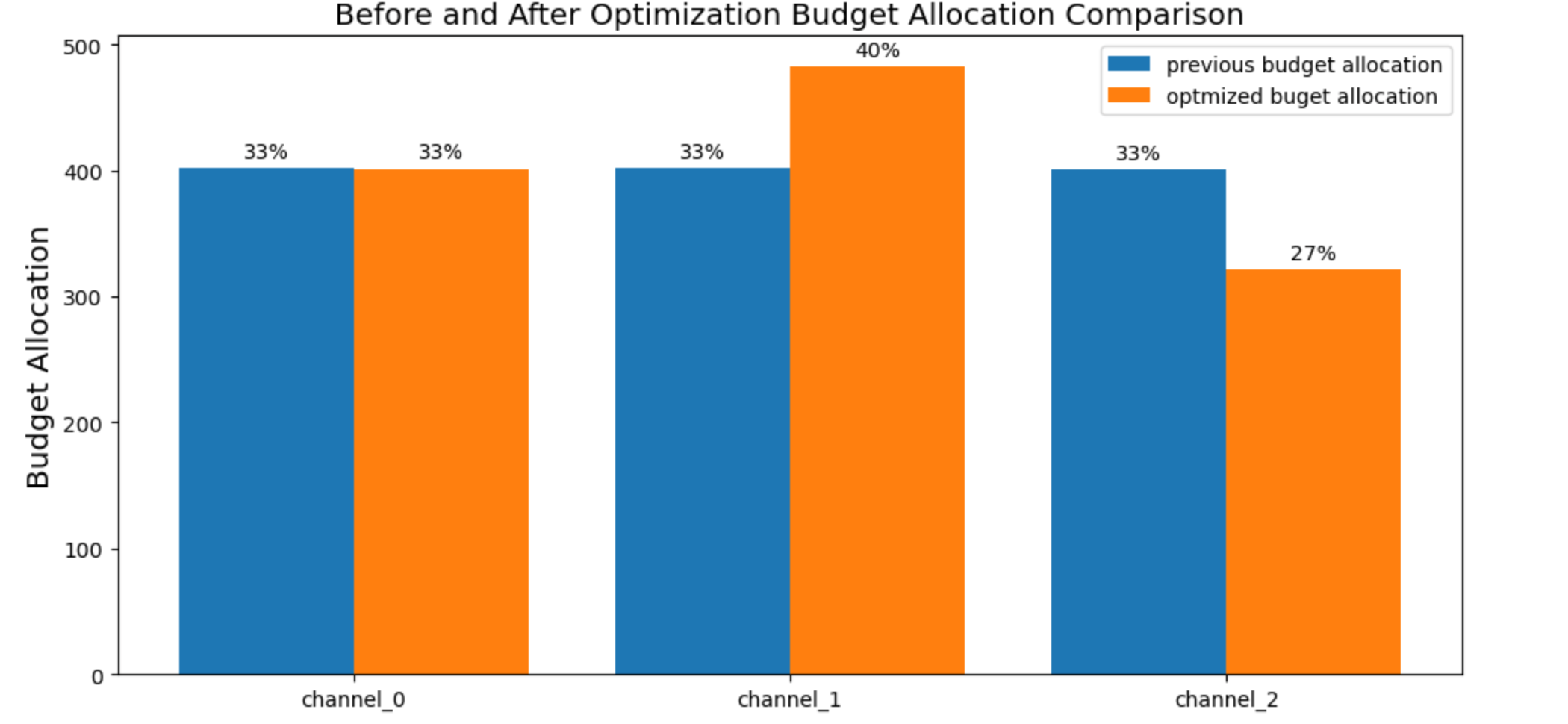
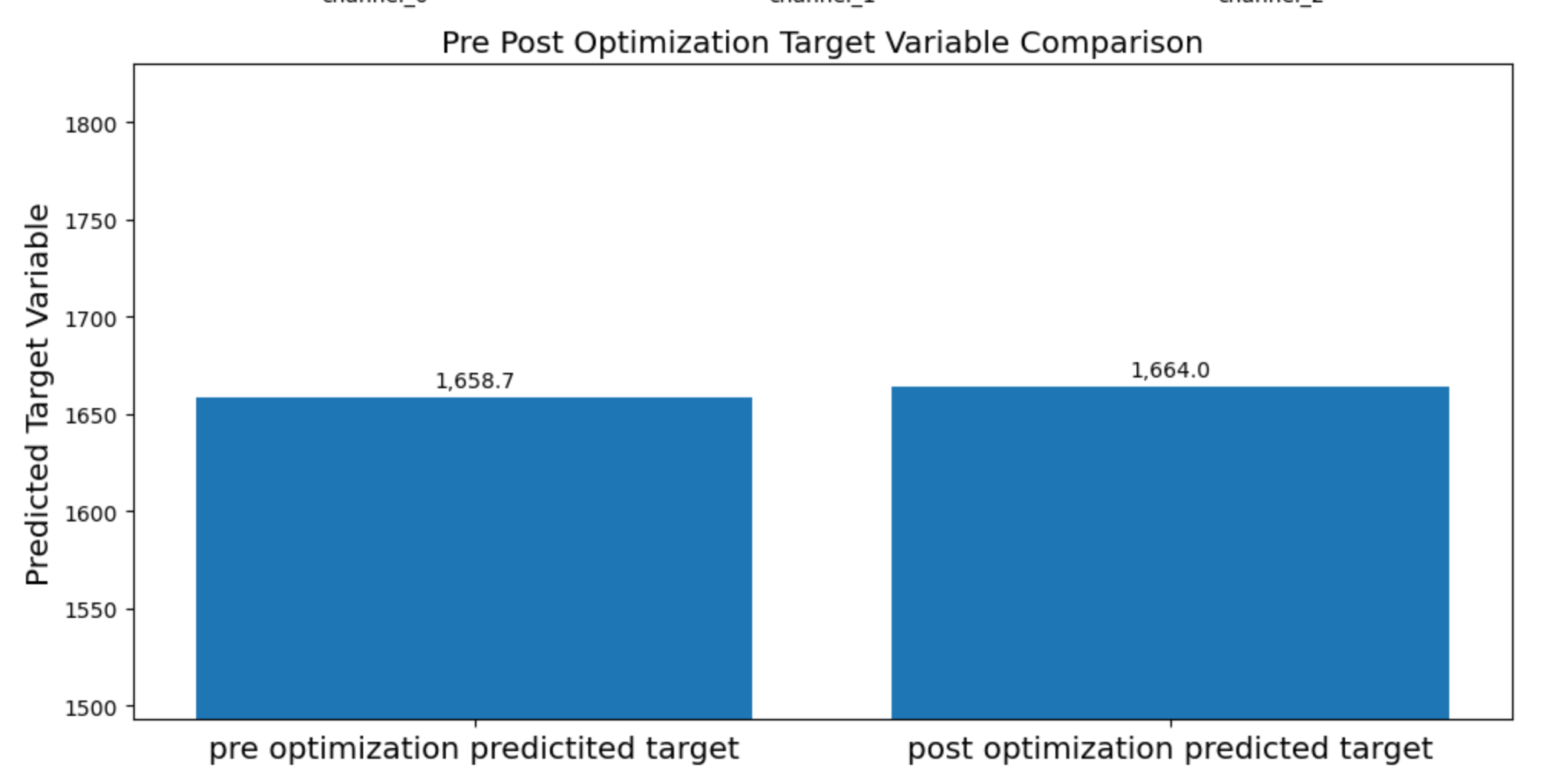
How reliable are the results?
It all depends on the data and how we configure the model and the decisions made by the data science team throughout the project impact the reliability of the results. They could be more or less reliable than a SaaS provider - you never know. One thing that’s great for both LMMM and RMMM is that they offer a train/test split (you train your data on a training set and then see how well it performs on a test set that it hasn’t seen before). Even though forecasting is not the goal in MMM, having some forecasting accuracy adds to the model's reliability.
To provide the best results, MMM calibration should be a standard practice once you implement MMM. Calibrating the LMMM is not easily done since there aren't any guidelines about how the model should be calibrated, so basically everything is left to the person who’s doing this project. But as mentioned above, this training/test set split can help people calibrate the results, as we can at least to some extent see how well the model performs so that adjustments can be made based on that. The main calibration in this model is adjusting priors / costs so that the output is reasonable. Moreover, the models should be monitored because things like COVID-19 can happen, and then some special treatment is needed. With SaaS, these steps are performed by your provider.
Once your model is up and running, your team will have to create plotted outputs from the data so that it is easy to visualize and understand the results. These are highly customizable depending on your scope, however, there are some differences between the LMMM and RMMM outputs.
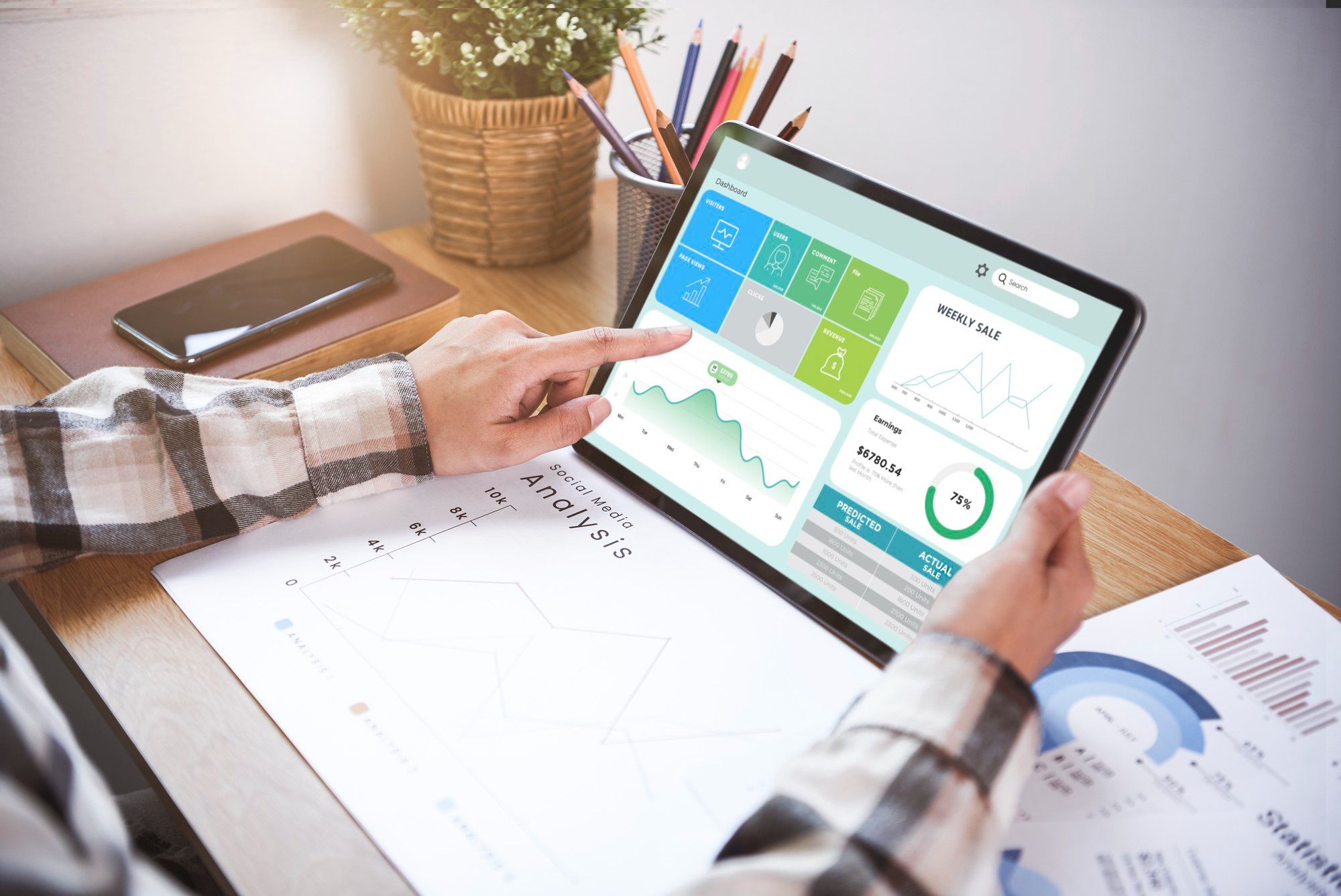
Limitations and Considerations for Lightweight and Robyn
Like any modeling solution, Lightweight MMM and Robyn MMM have their limitations. Here are some factors that users should keep in mind when evaluating these tools:
- Data quality: The accuracy and quality of the data used in marketing mix modeling is crucial to the accuracy of the results. If the data is incomplete, inaccurate, or inconsistent, the models may generate incorrect or misleading results. It is important to thoroughly clean and validate data before using either Lightweight or Robyn.
- Model assumptions: Both Lightweight and Robyn rely on certain assumptions about how the various marketing channels and factors influence sales. If these assumptions do not hold true for a particular business or market, the results of the model may be less accurate. Users should understand the assumptions and limitations of each tool and tailor the model accordingly.
- Interpretation: The results generated by marketing mix models can be complex and difficult to interpret, even with the simplified output formats provided by Robyn. Users should have a solid understanding of statistics and marketing concepts in order to interpret the results correctly and make data-driven decisions.
- Resource requirements: Both Lightweight and Robyn require significant resources to set up and run. Users will need access to data science expertise, as well as the hardware and software necessary to run the models. Additionally, marketing mix modeling is an ongoing process, so users should be prepared to invest resources in maintaining and updating the models over time.
- Scalability: Depending on the size and complexity of the business, the data sets used in marketing mix modeling can be quite large. Both Lightweight and Robyn may struggle to handle very large data sets, which could limit their usefulness for some users.
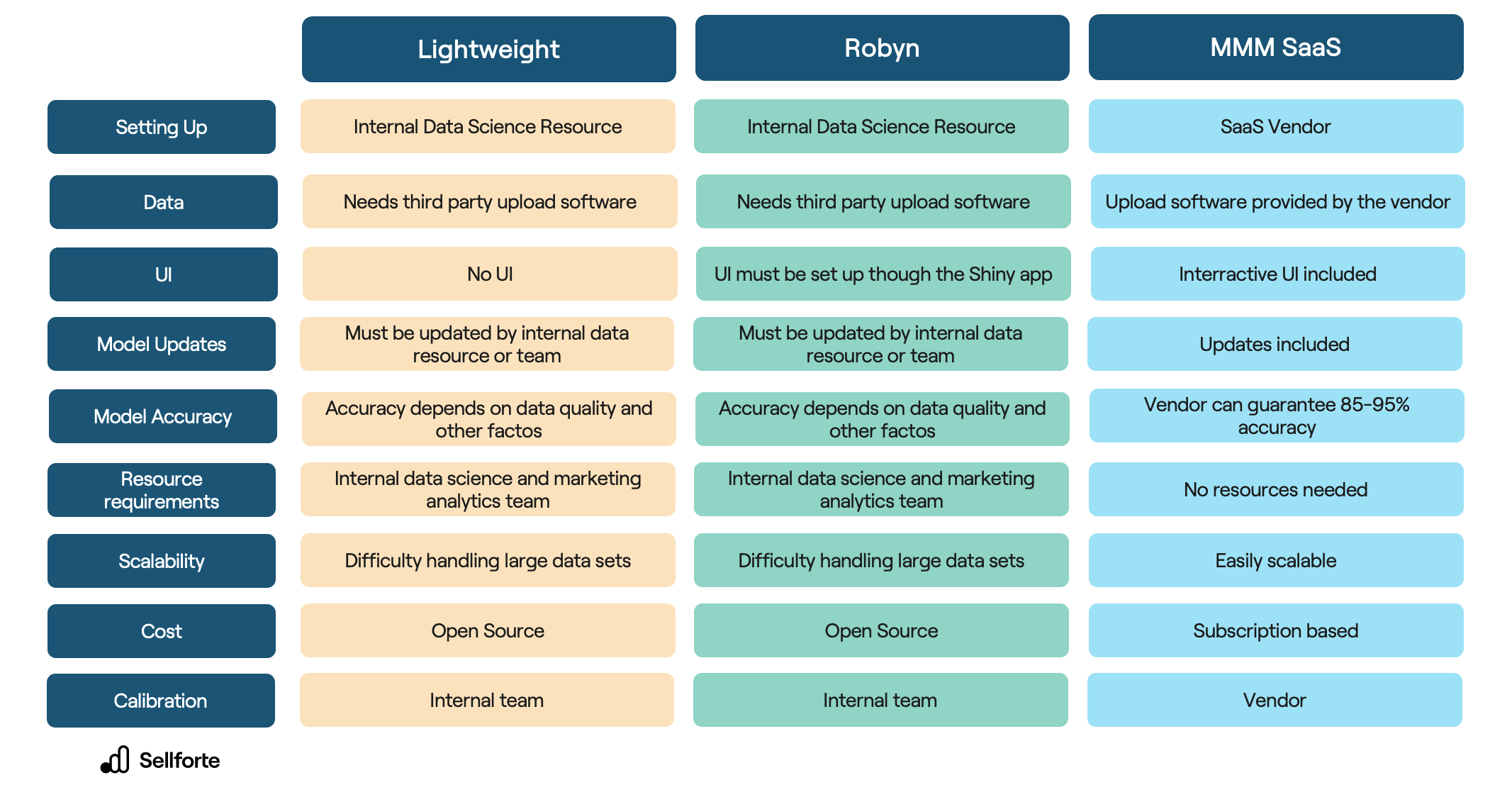
Which Marketing Mix Modeling approach should I choose?
Ultimately, it is up to you and your organization which MMM option you choose. However, we strongly recommend considering your skills as well as your resource availabilities before making a decision. Depending on the size and structure of your organization, you may opt for either an in-house MMM strategy or outsource to a specialized firm. An in-house MMM strategy allows you to maintain full control over the project and have complete oversight, while an outsourced approach could free up resources, give you access to specialized expertise and focus on core organizational tasks.
You May Also Like
These Related Stories
What is Causal Marketing Mix Modeling (MMM)?
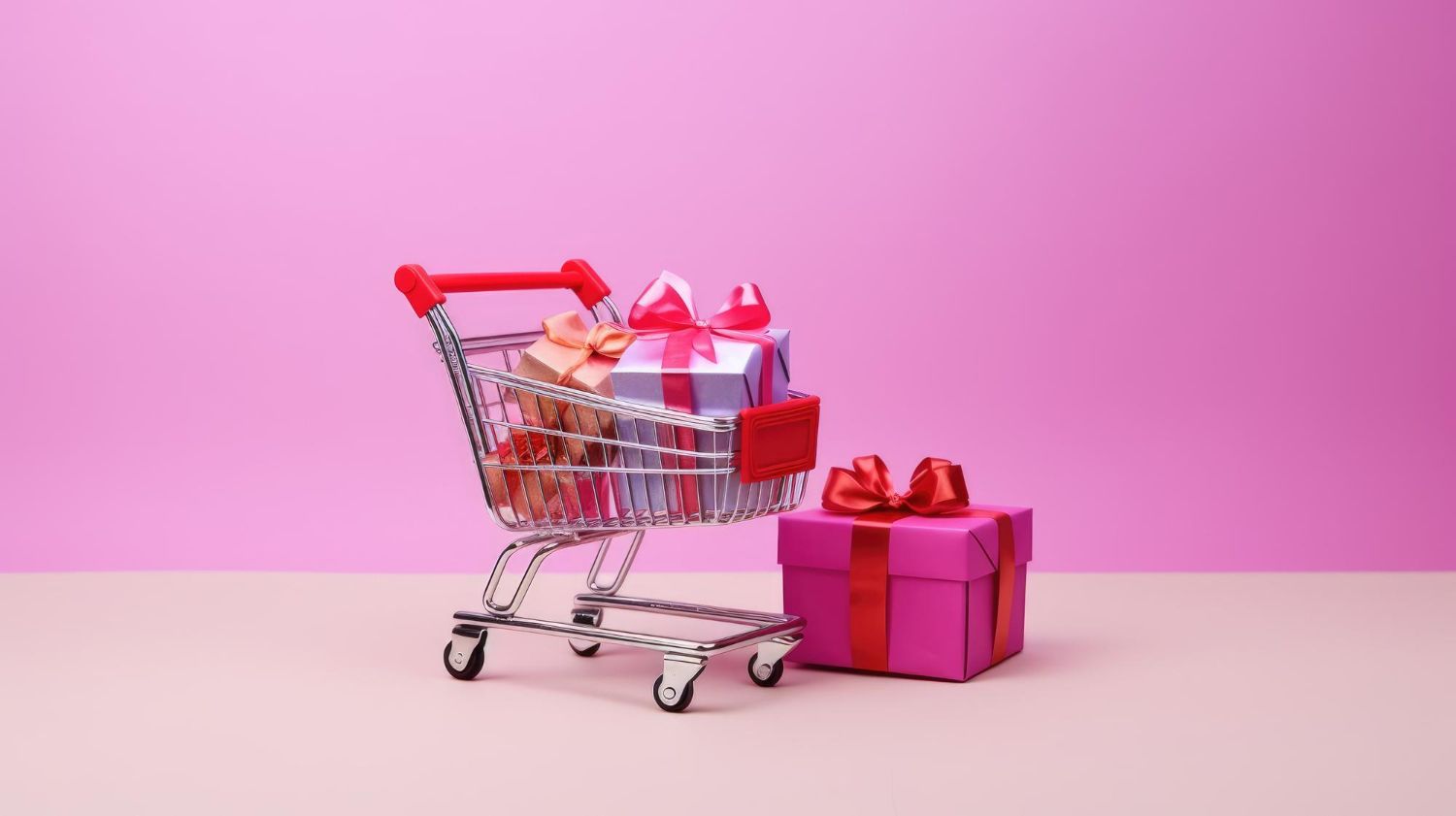
How Can eCommerce Brands Maximize Year-End Marketing Success?
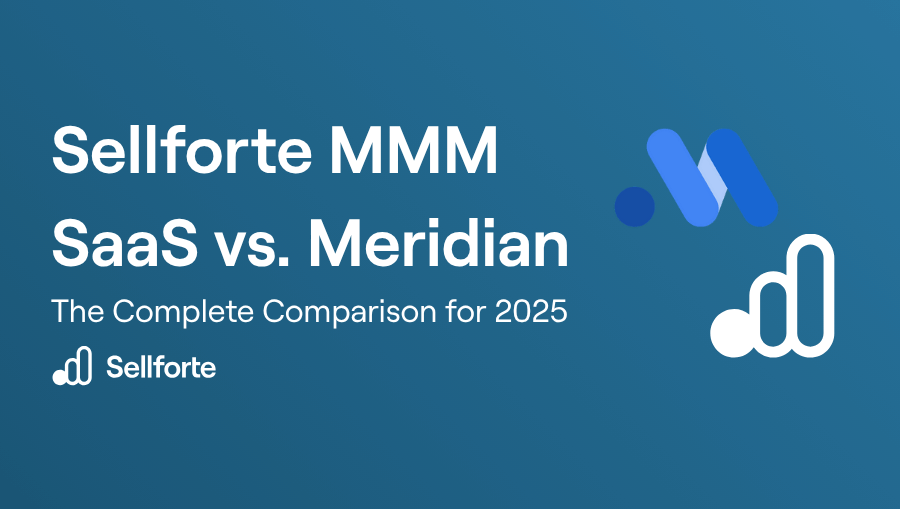