Blog
How to optimize Google and Facebook Ads spend?
May 24, 2023 | Paul Arpikari, Konsta Mustasilta, Carmen Bozga
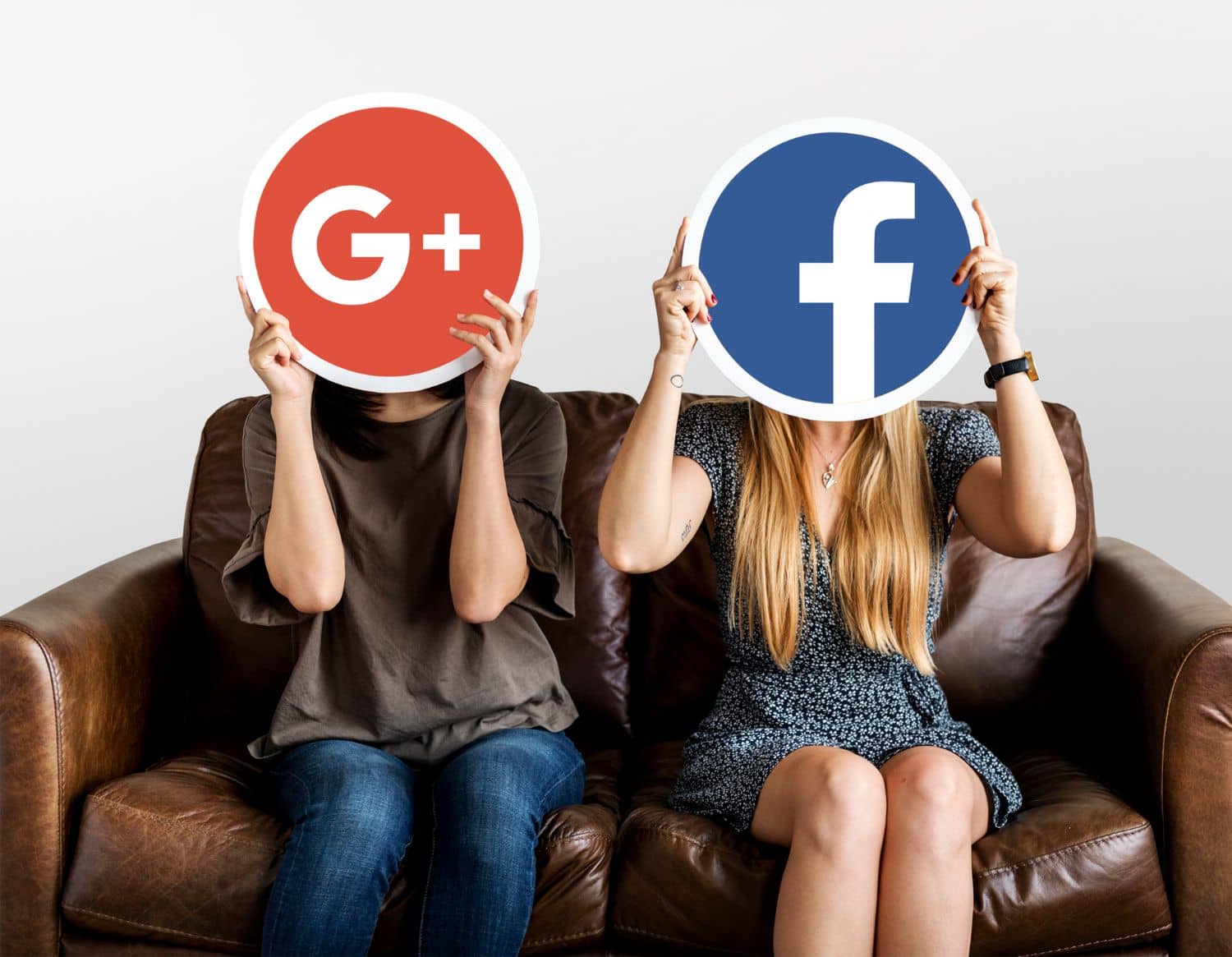
In today's ever-evolving digital landscape, it's no secret that advertising on platforms like Google Ads and Facebook Ads is crucial for businesses aiming to reach their target audience effectively. These platforms provide immense opportunities to showcase products or services to a wide range of potential customers. However, optimizing ad spend on these platforms can be quite challenging, and many advertisers struggle to accurately measure the impact and get the most out of their advertising budget. One of the primary obstacles they face is the attribution problem, where both Google and Facebook both claim credit for conversions, resulting in discrepancies in reported results. This article aims to shed light on the complexities of attribution and provide actionable strategies to optimize your ad spend more effectively.
The Attribution Challenge
Let's face it: the attribution problem is like the wild west of digital advertising. Both Google Ads and Facebook Ads tend to lay claim to the conversions, creating confusion and making it difficult for advertisers to determine the true impact of each platform. This leads to discrepancies when aggregating conversion data from both platforms and your organization's own data. Such discrepancies can mislead advertisers into making suboptimal decisions and hinder their ability to allocate resources wisely. In other words, it's like trying to hit a moving target while wearing a blindfold.
The Root Cause: Last Click Attribution and Buying Windows
To understand why attribution is such a challenge, we need to delve into the root cause of the problem. The measurement methodology employed by both Google Ads and Facebook Ads is based on Last Click Attribution, which attributes conversions solely to the last touchpoint or interaction before the conversion occurs. This approach oversimplifies the customer journey, disregarding the valuable influence of other touchpoints that may have played a significant role in driving conversions.
For example: "A customer sees an ad of a T-shirt in Facebook, but instead of clicking the ad, they search for that T-shirt brand from Google, after which they go and buy the shirt. In this case Google would get the conversion, even though the ad played in Facebook influenced the most to the buying decision.”
To complicate matters further, there are buying windows to contend with. These windows define a specific period within which conversions are attributed to a particular ad or touchpoint. If a user converts within the defined buying window after interacting with multiple ads from different platforms, each platform may claim credit for the conversion. As a result, advertisers end up with double-counting and inaccurate attribution, leading to a skewed understanding of their campaign performance.
Solutions for Optimizing Ad Spend
While the attribution problem may seem like a daunting challenge, there are practical strategies that can help advertisers navigate these murky waters and optimize their ad spend more accurately. Let's explore three approaches, each with varying levels of complexity, to find the one that best suits your needs.
Easy Route: A/B Testing - Putting Platforms to the Test
If you're looking for a simple yet effective way to tackle the attribution challenge, A/B testing might just be the answer. This approach allows you to compare the performance of two platforms by running ads exclusively on one while pausing the other. By isolating the impact of each platform, you can gain valuable insights into their individual effectiveness.
Here's how you can implement A/B testing in a few simple steps:
- Select the platforms: Choose the two platforms you wish to compare, such as Google Ads and Facebook Ads.
- Define test groups: Create two distinct groups of users - Group A and Group B. Group A will be exposed to ads on Platform A (e.g., Google Ads), while Group B will be exposed to ads on Platform B (e.g., Facebook Ads).
- Implement the test: Run the ads for a predetermined period, ensuring that the test groups are exposed exclusively to their respective platforms.
- Measure and analyze results: Compare the performance metrics of each group, such as conversions, click-through rates (CTR), cost per acquisition (CPA), and return on ad spend (ROAS). Analyze the data to identify which platform delivers better results.
Semi-Easy Route: Lift Test Studies - Measuring Incremental Impact
Another strategy for optimizing ad spend is to conduct lift test studies. These studies aim to measure the incremental impact of advertising on conversions, going beyond the organic or baseline performance. By comparing the lift generated by Google Ads and Facebook Ads separately, advertisers can allocate their budget based on the platforms' respective contributions, resulting in more accurate attribution.
Here's how to conduct a lift test study:
- Define control and exposed groups: Split your target audience into two groups - a control group and an exposed group. The control group is not exposed to any ads, while the exposed group is shown ads on the platforms you want to test.
- Run the ads: Implement your advertising campaigns on the chosen platforms, ensuring that the exposed group receives the intended ad exposure.
- Measure conversions: Track the conversions in both the control and exposed groups during the campaign period.
- Calculate lift: Compare the conversion rates between the control and exposed groups to determine the incremental impact of the ads. This lift represents the additional conversions generated by the advertising campaigns.
- Analyze the results: Evaluate the lift generated by each platform to understand their individual contributions. Consider factors such as the magnitude of lift, statistical significance, and the cost of running the campaigns. This analysis will help you determine which platform provides a higher return on investment and should receive a larger portion of your ad spend.
By leveraging lift test studies, advertisers can gain insights into the true impact of their advertising efforts on conversions. This approach helps address the attribution problem by quantifying the incremental lift provided by each platform, allowing for more accurate attribution and informed decision-making in budget allocation.
Hard Way: Media Mix Modeling (MMM) - Statistical Analysis for Optimal Investment
For advertisers seeking a more comprehensive approach to optimize their ad spend, media mix modeling (MMM) provides a sophisticated statistical analysis of the performance of media investments. MMM considers various factors such as ad spend, reach, frequency, seasonality, and external variables like economic conditions to determine the effectiveness of different advertising channels, including Google Ads and Facebook Ads.
Here's how to implement media mix modeling:
- Gather historical data: Collect data on past advertising campaigns, including ad spend, impressions, reach, conversions, and any other relevant metrics. Additionally, collect data on external factors that might impact conversions, such as economic indicators or seasonal trends.
- Build the model or use a SaaS provider: Utilize statistical techniques to analyze the historical data and develop a media mix model. This model will estimate the impact of each advertising channel on conversions, considering the interplay between different channels.
- Calibrate the model with lift test data: Incorporate the results from lift test studies conducted on Google Ads and Facebook Ads to refine and calibrate the media mix model. The lift test data provides actual lift figures and helps validate the accuracy of the model's estimates.
- Allocate ad spend: Utilize the calibrated media mix model to determine the optimal allocation of your ad spend across different channels. The model will provide insights into how much budget should be allocated to Google Ads and Facebook Ads based on their estimated contributions to conversions.
Media mix modeling allows advertisers to take a holistic approach to optimize their ad spend by considering the effectiveness of various advertising channels and their synergistic effects. While it requires more expertise and resources to implement, media mix modeling provides a robust framework for making data-driven decisions in ad budget allocation.
Curious to learn more? Book a demo.
Related articles
Read more postsNo items found!