Blog
Why should I use Marketing Mix Modelling when I have Google Analytics?
November 29, 2022 | Chris Kervinen
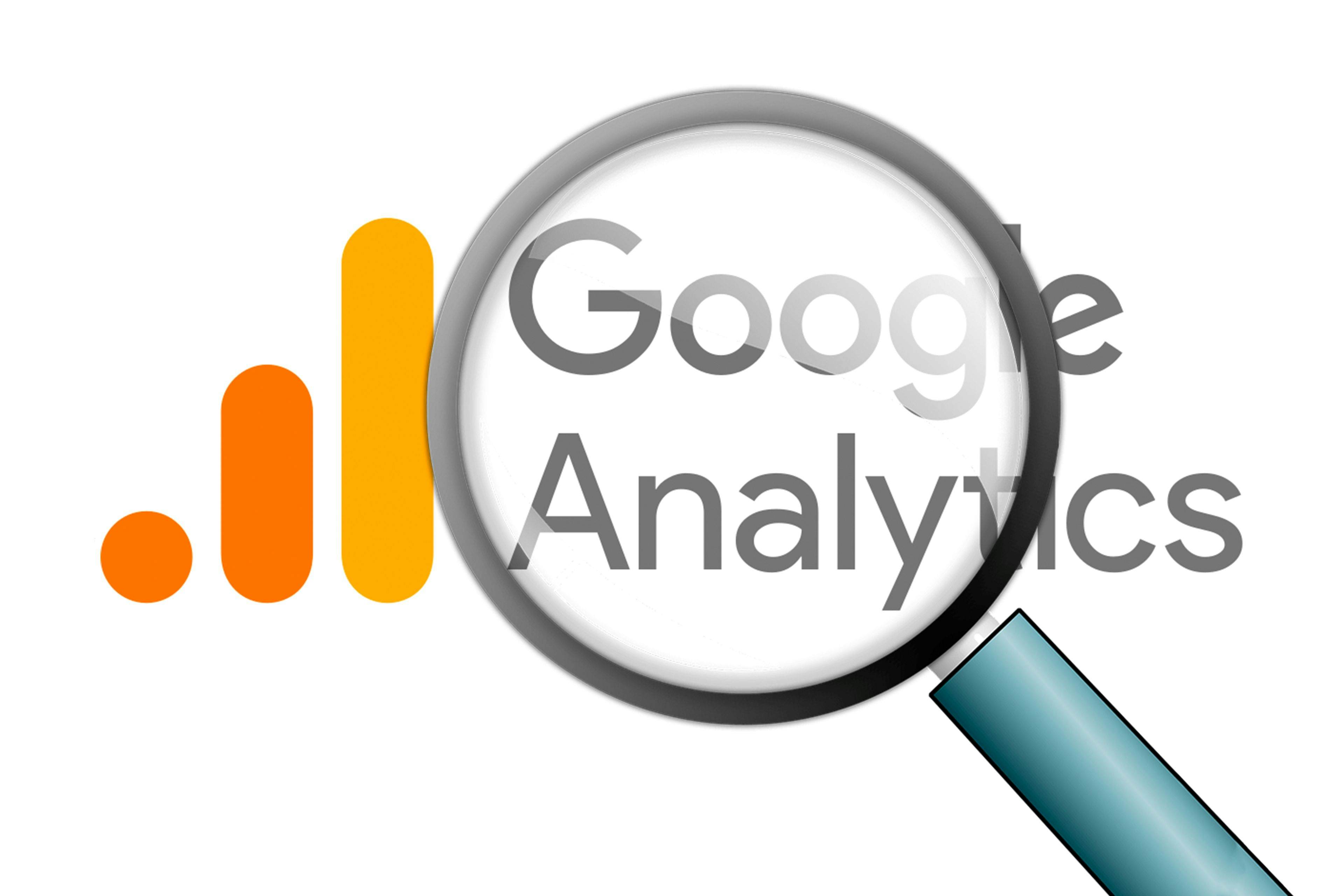
Google Analytics has been the #1 tool to track how different campaigns and channels are driving conversions. It should not, however, be the only source of truth when planning your next digital campaign.
As a digital marketer, you’re more than familiar with Google Analytics (GA) and its capabilities. For years GA has been the go-to source for insights regarding website visits and digital sales, but the latest changes in both EU’s privacy regulation and consumers’ view on cookies have presented major challenges for the tool.
The question is, how accurate Google Analytics results are? And how accurate they will be in the future?
User blocks cookies = no results
Simply put, GA (both Universal Analytics & Google Analytics 4) cannot process data from users that have rejected cookies. This includes also various Ad Blockers that stop the GA by default (e.g. uBlock Origin, Purify & AdBlock Browser), as well as Ad Blockers that can be configured to block cookies (such as AdBlock & Ghostery).
Statista report shows that there were 763 million AdBlock users worldwide in 2019, and the number has grown steadily over time.
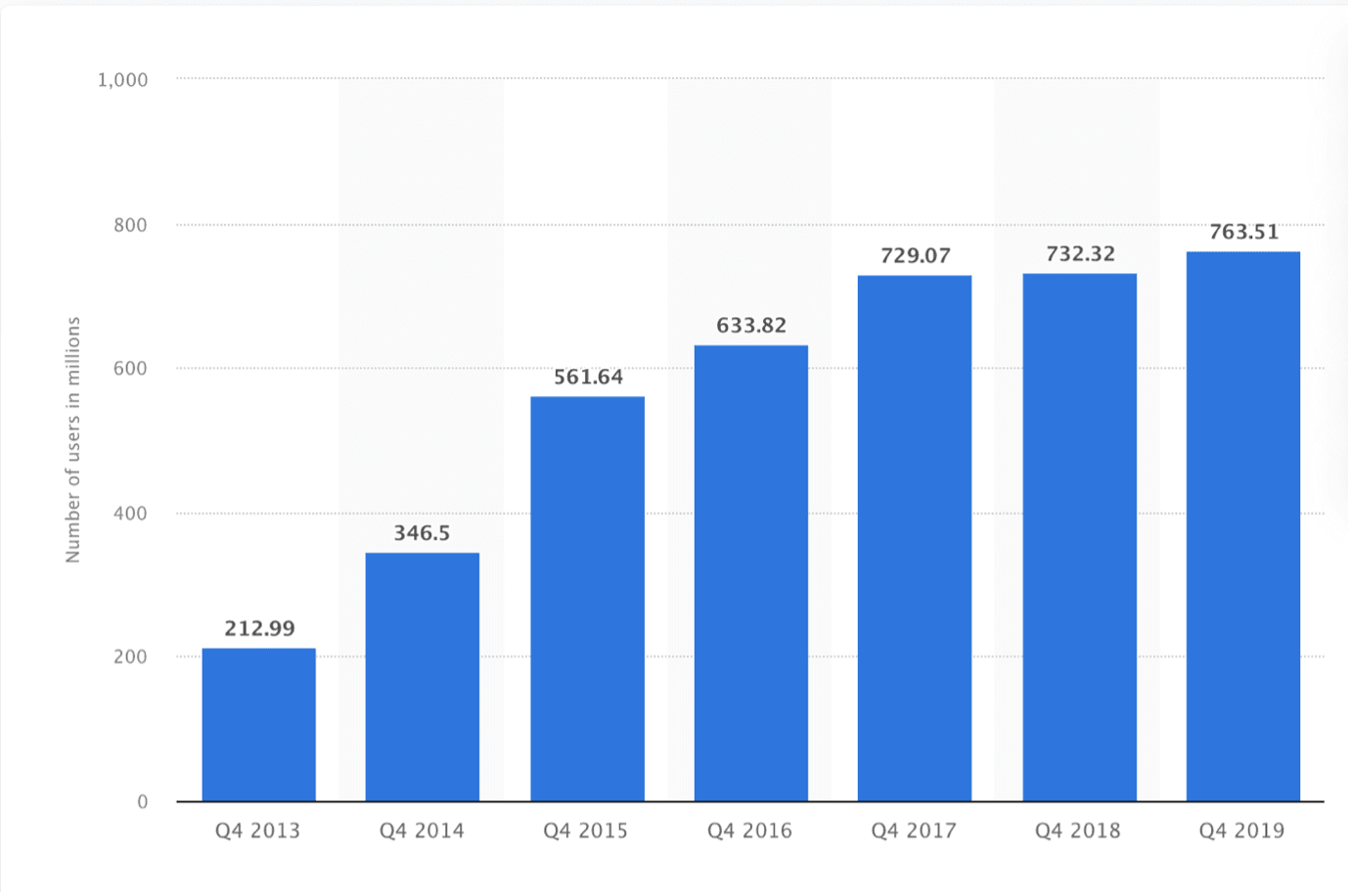
Cookie rejection is first and foremost a problem for European marketers, as according to GDPR provisions sites using GA products must display a cookie consent banner. Failing to do so can lead to a fine of up to €10 million , or 2% of the firm’s worldwide annual revenue.
First world problems
Combining the increasing amount of AdBlock users worldwide with the strict GDPR regulations within the EU leads to the fact that GA can’t and doesn’t report European traffic accurately. A concrete example of GA’s futility within the EU is Austria and France’s decision to rule GA illegal under GDPR.
“A concrete example of GA’s futility within the EU is Austria and France’s decision to rule GA illegal under GDPR.”
Not being able to capture most of your website traffic is problematic as is, but there are various other repercussions as well:
1. GA is missing digital mediums from the awareness and consideration stages
Attribution modeling is heavily dependent on data about which touchpoints were visited on the decision-making journey. Without consumers’ consent on cookies, there’s no way to track the digital journey across various sites and platforms. And without data on which touchpoints were visited by a certain individual earlier, GA must opt for last-click attribution – which paints a quite distorted picture of how marketing is driving results (typically the interest and need have been sparked way earlier than at the point of purchase).
While the importance of Awareness- and Interest -mediums may vary between different industries, no company can afford to neglect them when making decisions about budget allocation:
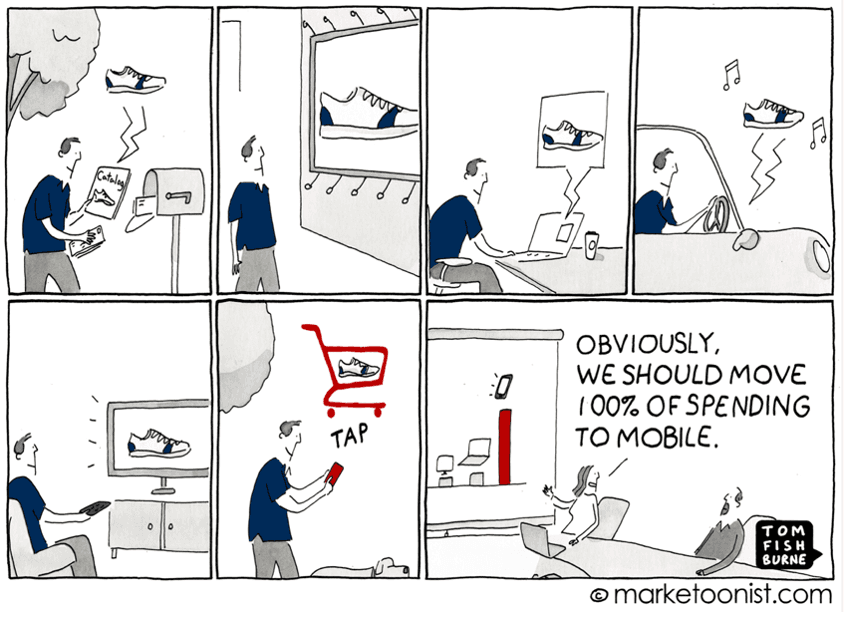
The same issue has characterized offline media measurement for years, and for the very same reason (in offline there are no traceable touchpoints between the mediums, just as there are none after cookies depreciate in digital marketing). And just as in offline, digital mediums can and should be modeled with Marketing Mix Modelling in order to get reliable results.
2. Google tries to close the gaps with Machine Learning and Blended Data
This sounds very cool and futuristic, but in practice, this means that Google’s algorithms are extrapolating results based on data subsets, which isn’t cool and futuristic at all. The whole point of data analytics is to derive insights from the entire dataset, so running the modeling with partial datasets includes a high risk of bias in the results.
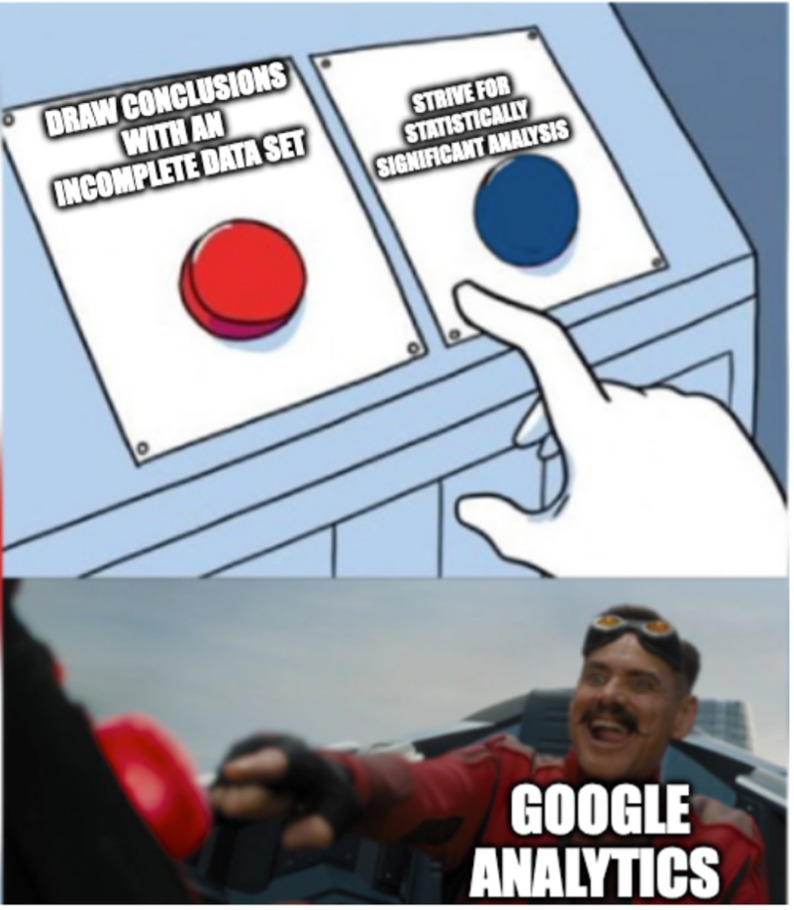
3. It is really difficult to optimize your digital budget allocation with siloed insights
GA, just like Apple, Meta, and other ad networks, uses a walled-garden approach to its online advertising business, meaning it provides analytics and optimization tools for Google’s channels and these channels alone. Google won’t ever recommend investing part of the budget in Meta or vice versa, even though it would be optimal for the marketer. Trusting that the platform can provide you with the best advice with partial data and no visibility on what’s happening on other ad networks is risky. Throw in the fact that the platform tries to sell you as much media as possible, and you might start to demand more transparency into what’s happening within GA.
“Trusting that the platform can provide you with the best advice with partial data and no visibility on what’s happening on other ad networks is risky.”
In a nutshell, using GA as the only tool to understand your online statistics is suboptimal, to say the least. Instead, digital marketers (especially within the EU) should look for solutions that are:
- 🇪🇺 GDPR-compliant (or in other words, privacy-friendly tracking & measurement),
- 🔬 Scientifically solid (or at least methods that aren’t black box solutions) and
- ⚖️ Objective/unbiased/trustworthy
Why you want to use Marketing Mix Modeling alongside Google Analytics
The underlying problem with GA is data: What data goes in, how this data is modeled, and what the output data tries to tell us.
What data goes in
As mentioned above, data that goes into GA is incomplete – the model doesn’t see for example the activities on other ad networks. Moreover, you can’t really include external data sources, such as Covid-19, macroeconomic or weather data, which can have a substantial effect on the outcome. Neglecting business-critical datasets lead to overvaluation in the modeled metrics, which is one of the key reasons ad networks can’t measure the baseline accurately.
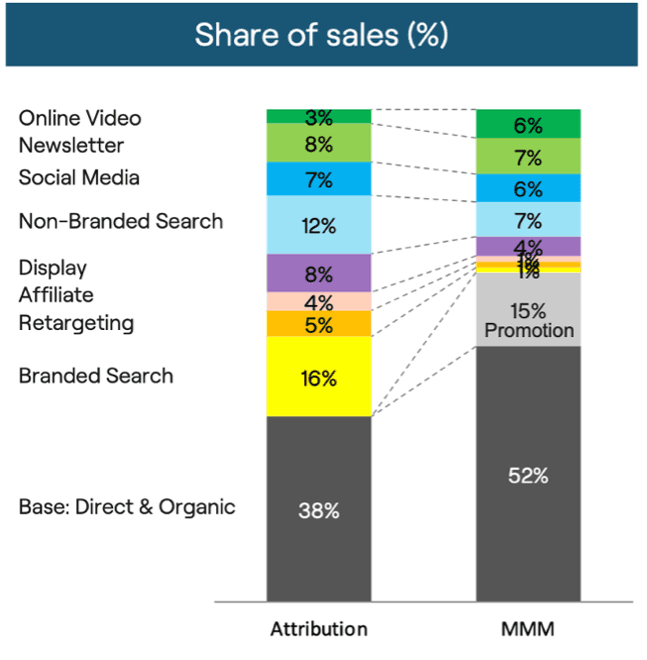
Marketing Mix Modeling enables the user to leverage marketing data from all ad networks and model each medium’s contribution to website conversions. Furthermore, users can include external data sources to help the model to measure the baseline more accurately in different scenarios (a simple example would be including weather data when modeling ice cream demand. Without adding it media uplift would be overvalued on sunny days).
How this data is modeled
Due to the latest privacy regulations and the gaps in the input data, GA(4) relies on machine learning data sampling to make up for the data loss. Again, sounds very cool and futuristic, but in practice, GA can extrapolate the results after a data cap of 500K website visits. This means if you would collect 5 million page views, the data for the remaining 4,5M views is an assumption based on the 500K sample. Not very cool and futuristic after all.

Google is, however, making efforts to become more scientific. After Apple’s iOS14 update and Facebook’s release of the Robyn Media Mix Modeling tool, Google has been developing its own MMM solution, namely LightweightMMM . It’s good to keep in mind that this is a full open-source python library, which requires technical know-how to be implemented in any meaningful way.
Safe to say that Marketing Mix Modeling is the methodology of the future.
What the output data tells us
At the end of the day, we’re all very busy people, and if you’d have to prioritize one thing in your analytics, it should probably be the outcome of the analysis. GA tells us a pretty one-sided story of how your marketing is performing, leaving out important factors such as other ad networks and external data sources.
No modeling is 100% accurate, of course, as marketing is one of the most complex functions in any company. You should still validate different solutions based on the objectivity and the scientific aspects of different options to avoid hasty decisions.
Circling back to the leading principles for analytics solutions:
- GA cannot be objective as Google sells the media which effectiveness it also models.
- GA operates with incomplete datasets and fills the gaps with data sampling, which isn’t scientifically kosher.
- GA and GDPR cannot coexist in any functional way. Siding with GA leads to a hefty fine, sooner or later.
Interested to try Marketing Mix Modeling? Sellforte provides user-friendly & browser-based Marketing Mix Modeling Software-as-a-Service for digital marketers operating within the B2C sector?
Curious to learn more? Book a demo.
Related articles
Read more postsNo items found!