Meta MMM Summit 2022 from MMM SaaS provider's perspective
What an event!
Last Wednesday it was again time for Meta’s annual global Marketing Mix Modeling Summit to hear about new methodologies, calibration techniques, innovation, open source solutions, and new industry product offerings.
And we were, of course, quite excited about it.
Marketing measurement is changing big time
One of the most important presentations of the day was from Meta's Kate Guimaraes and Vinh Mai. They introduced the changes happening in the ad ecosystem and how advertisers have reacted to the measurement challenges recently.
We heard that advertisers are currently adopting different marketing measurement tools as a response to GDPR/CCPA, Apple iOS14 privacy changes and deprecation of 3rd party cookies from browsers.
Marketing Mix Modeling (MMM) has now become the #1 tool that advertisers have adopted as a result of changes in the ad ecosystem. We learned that Meta is supporting a diverse network of providers to build a low-cost fast turnaround MMM SaaS solution.
The biggest gap is in the supply
During the introduction following graphs were presented:
- Showing the rise of MMM as a measurement tool
- The gap in supply to meet the rising demand.
The message was to point out that even though there are many traditional MMM providers on the market, many of them have been born to serve offline advertisers and large brands that have had this problem for decades. Based on our own experience, these providers include traditional consulting companies, research companies and media agencies' analytics arms.
The problem with traditional MMM consultancies and media agencies is that the cost of service is high and the frequency of updates is low. Therefore, until this day MMM has been available only to the largest advertisers who can afford mammoth projects and high running costs of these custom-built, heavy solutions. Also, the update frequency of these solutions (typically once, twice or four times a year) is not high enough for the digital teams requiring daily or weekly updates to their budget allocation.
Meta sees that for the market to change and for everyone to get the benefits of MMM, new easy-to-implement solutions need to be born. These solutions should be much more automated and standardized, resulting in more affordable costs and faster update frequency of results.
What Meta is expecting from the Marketing Mix Modelling market?
After highlighting the gap in the market Meta summed up what they are looking forward to seeing from the market in the future. There were five main categories for MMM SaaS solution:
MMM SaaS provider’s view on these 5 topics
As Sellforte set up the MMM SaaS strategy & priorities already back in 2018 and sees the world similarly in many ways, we decided to go through these five topics from our point of view:
1. Low cost
The biggest cost of MMM projects comes from human labor. If the provider does not have a real automated product, then they need to rely on manual work in data collection, data cleaning, data validation, model configuration, results validation, results visualization (PowerPoint) and creation of insights (by consultants).
MMM platforms such as Sellforte help bring the costs down by automating the data processing and modelling while providing standard views for visualization of the results and creating insights. Why should you invent the wheel again in every MMM project when you can incorporate your learnings into an automated system and reap the rewards?
2. Speed & Frequency
Having access to up-to-date data and results is critical for adopting a true test-and-learn culture. Besides lowering the cost and barrier of entry, an automated MMM platform allows to ramp up the speed of insight delivery by refreshing the data and the results more frequently – even daily or weekly.
The speed should not come at the cost of quality. The modelling methodology and data pipelines need to be robust enough to avoid having to babysit them and consultants to tweak the results. On the other hand, automated systems are rarely fully fire-and-forget: there needs to be continuous monitoring of the system so that any issues e.g. in model training are flagged for the responsible team to address them swiftly.
3. Agility
Based on our experience, you only realize the potential of MMM once you have started using it. That is why it is important to allow the users to re-configure their data and models to serve their needs better.
Again, automation helps here a lot. It is especially crucial to have good data integration tools. They should allow adding new fields to the input data flexibly and connecting new sources with minimum effort. Otherwise, the inertia of the system slows you down too much for you to try out new things.
4. Transparency & Trust
The simple underlying mechanism makes cookie-based attribution models easy to understand and trust – so easy, in fact, that the apparent shortcomings are often forgotten. On the contrary, MMM models can be quite complex mathematically, relying on arcane technologies such as probabilistic machine learning.
The difference in the underlying technology makes it even more crucial for MMM platforms to be transparent and gain the trust of the customers. The best way to earn the trust is to provide real measurable improvements to marketing effectiveness, but to get that far the platform needs to provide transparency on the whole process from the data processing to the modelling methodology to the outputs.
When developing or applying any kind of ML models, it is all too easy for data scientists to get lost in the technical details – MAPEs, LOOs, and whatnot – while forgetting the business validation of models. Business validation does not have to live outside the computational machines: it can be built into assumptions that serve as the very foundation of the models.
Meta’s open-source solution, Robyn from Igor Skokan and the team offers a fantastic example of this by favoring solutions where the share of incremental sales for each media channel is close to its share of the investment budget. It also enables calibrating the results based on ground truths such as lift tests, which is essential for both building trust by improving result quality and consistency.
The need for transparency is one of the key reasons why black box models like neural networks are not our tools of choice for MMM.
5. Usability
Even MMM with daily updates and pinpoint accuracy is a waste of time without clear insights. For a real scalable MMM SaaS, these insights should be gained by the users themselves easily from the tool, not delivered by a consultant in a PowerPoint deck after exhaustive ad-hoc analysis.
MMM SaaS solution lets user go beyond high-level results and find themselves the insights
Also, we have not yet met a data scientist who loves to take screenshots from their Python or R graphs and paste them to a PowerPoint deck for the marketing team’s users. Therefore, it is of utmost importance to provide an easy-to-use interface for the marketing team’s users which allows access to the results 24/7 with summary views, drill down options and self-service tools for planning scenarios.
Final words
It really seems that the marketing measurement industry is moving on fast now and we can witness during the 2020s the rise of MMM SaaS solutions.
Thank you Meta for the great event! We are very much looking forward to the MMM summit in 2023 and seeing where the MMM SaaS market has progressed by then.
Curious to learn more? Book a demo.
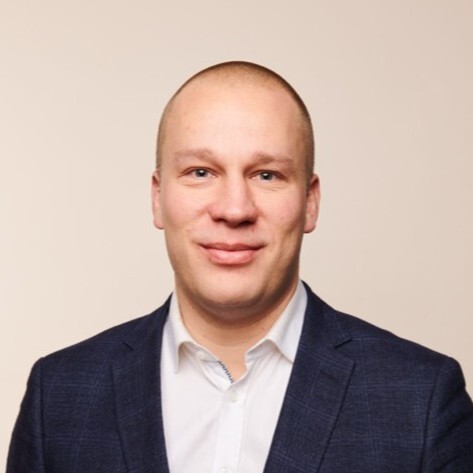
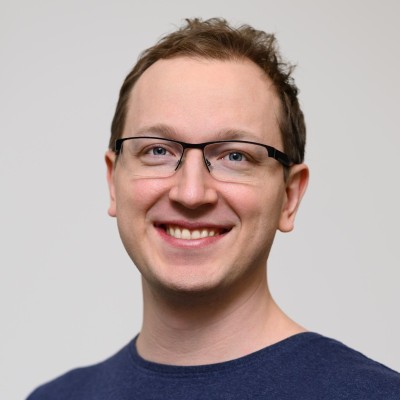
You May Also Like
These Related Stories
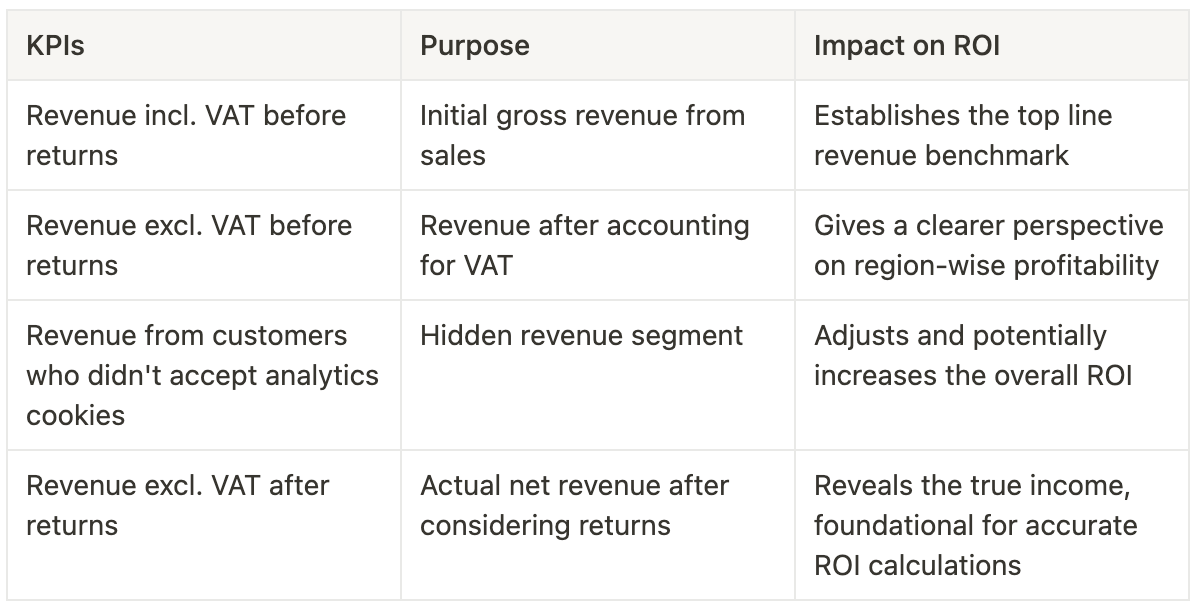
How Can MMM Help Optimize eCommerce Revenue Metrics?
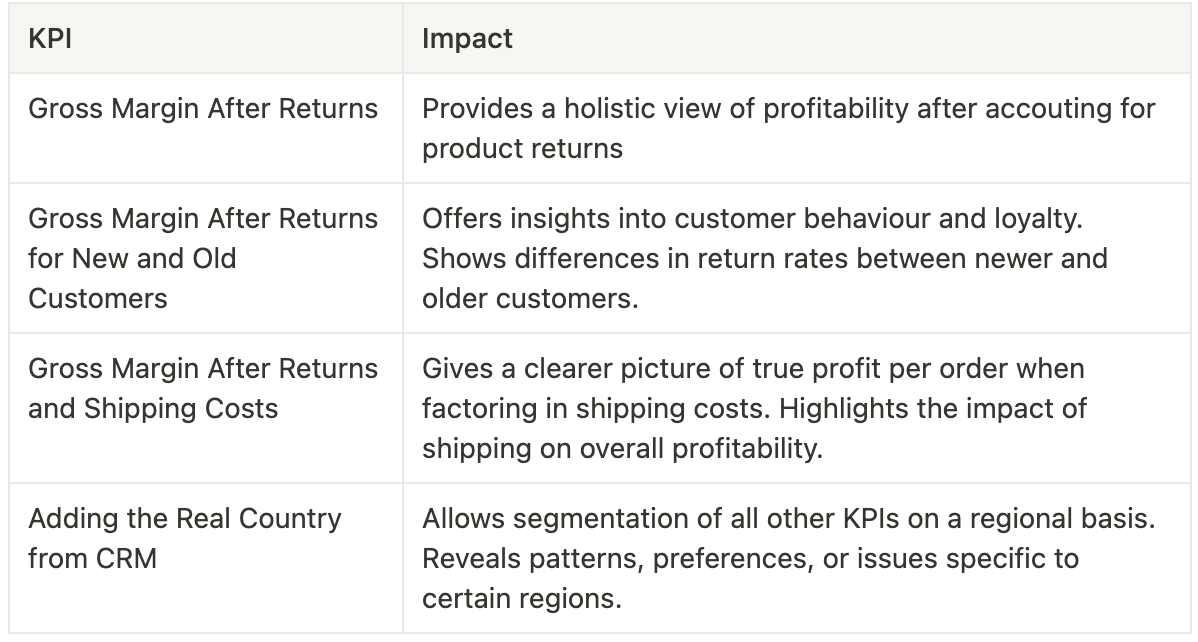
Why optimize eCommerce Profitability?
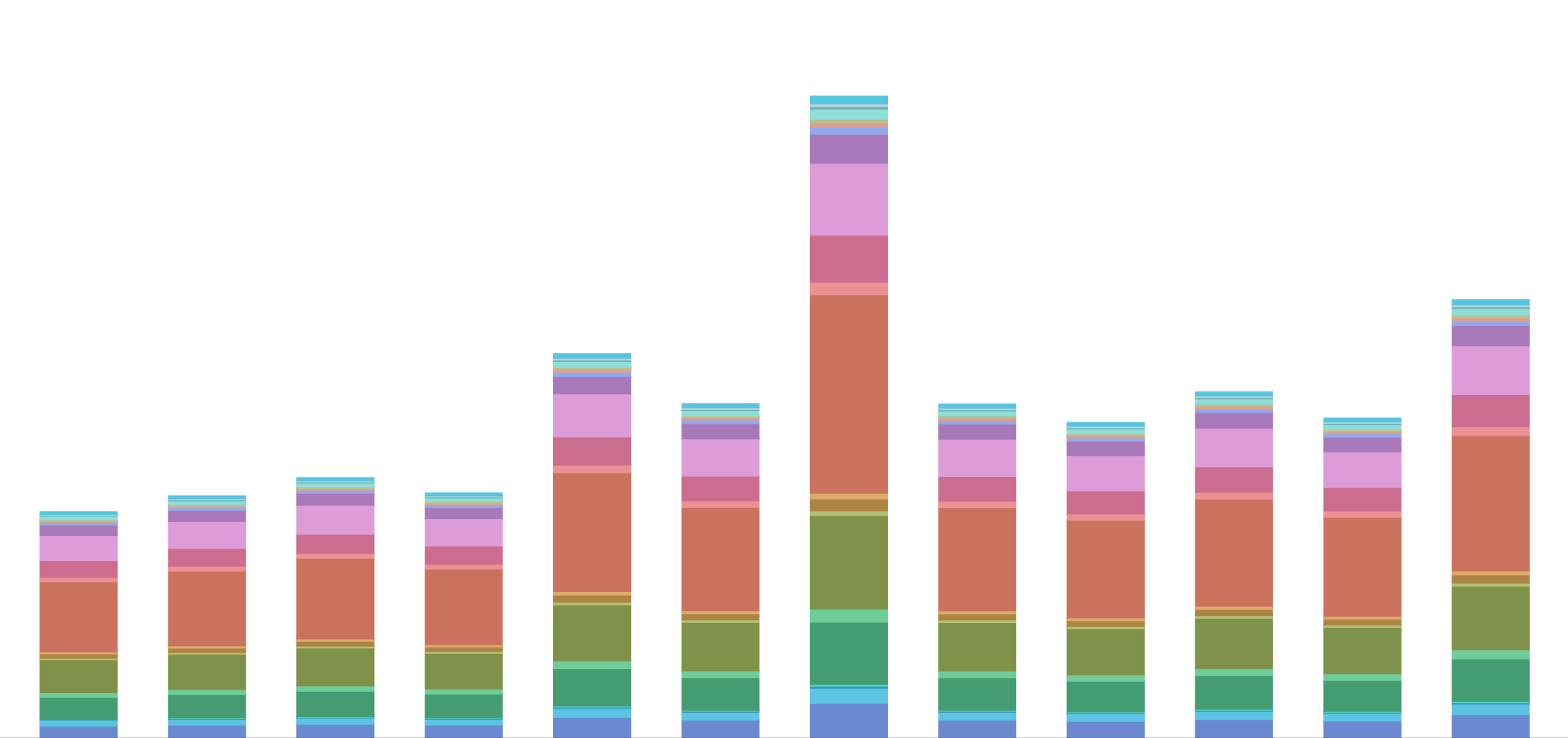