Causal Marketing Mix Modeling
Sellforte's Next Generation Marketing Mix Modeling technology ensures you will get high quality MMM results, which you can trust. We leverage Bayesian modeling, the golden standard in MMM, and calibrate models with incrementality tests and attribution data.
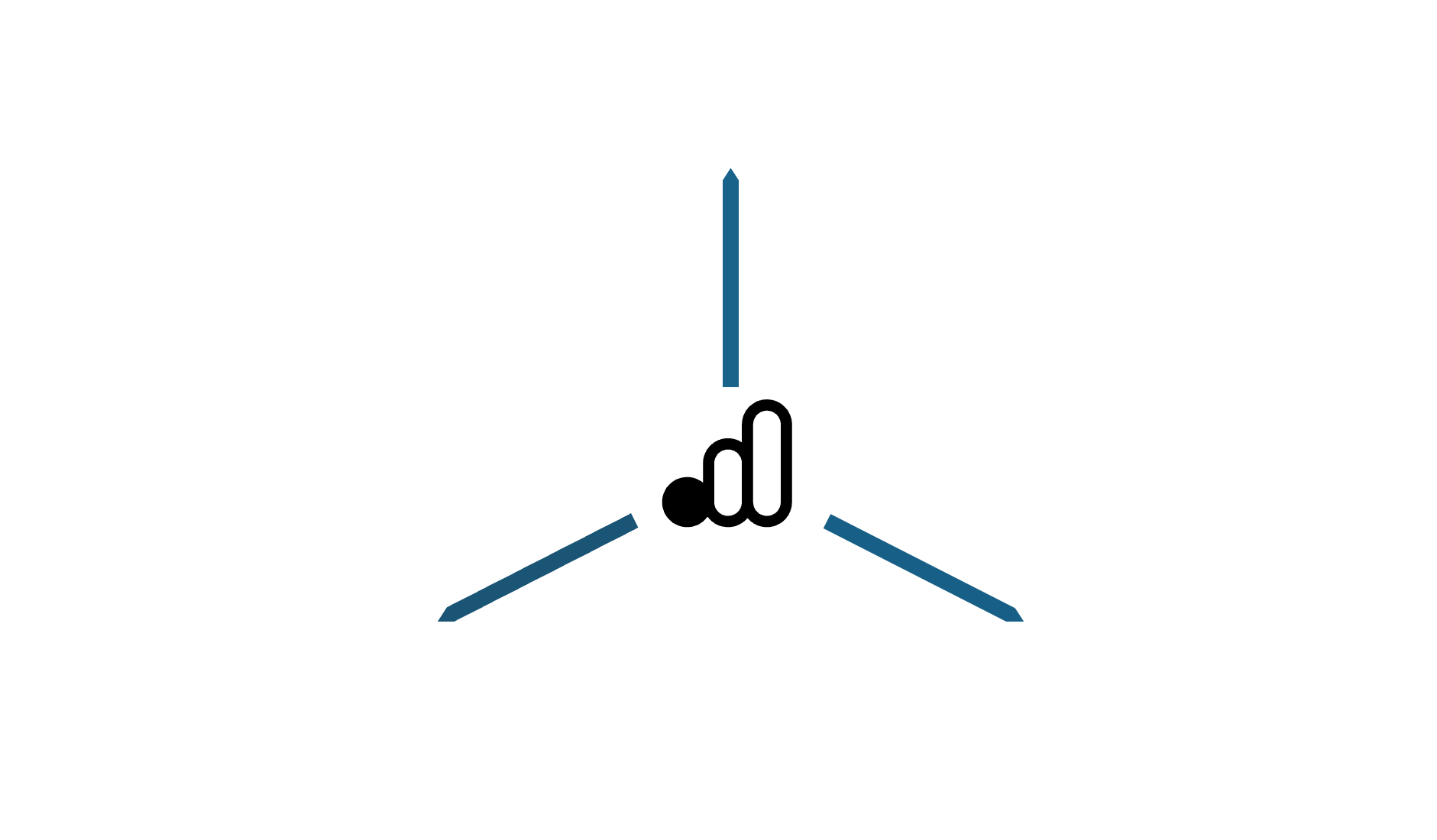
Marketing Mix Modeling in a nutshell
Marketing Mix Modeling, or MMM, is an approach for estimating marketing’s ROI through time-series analysis. The model learns sales impact of each channel by analysing historical data on marketing activities and sales. As an output, MMM provides
- Base sales: Sales you would get without marketing
- Incremental sales driven by marketing
- Incremental sales driven by promotions
- ROI and response curve for each marketing activity
Learn more about MMM here. Sellforte’s Next Generation MMM solution leverages model calibration with incrementality tests and attribution data. This enables drastically more reliable and granular results compared to traditional MMMs.
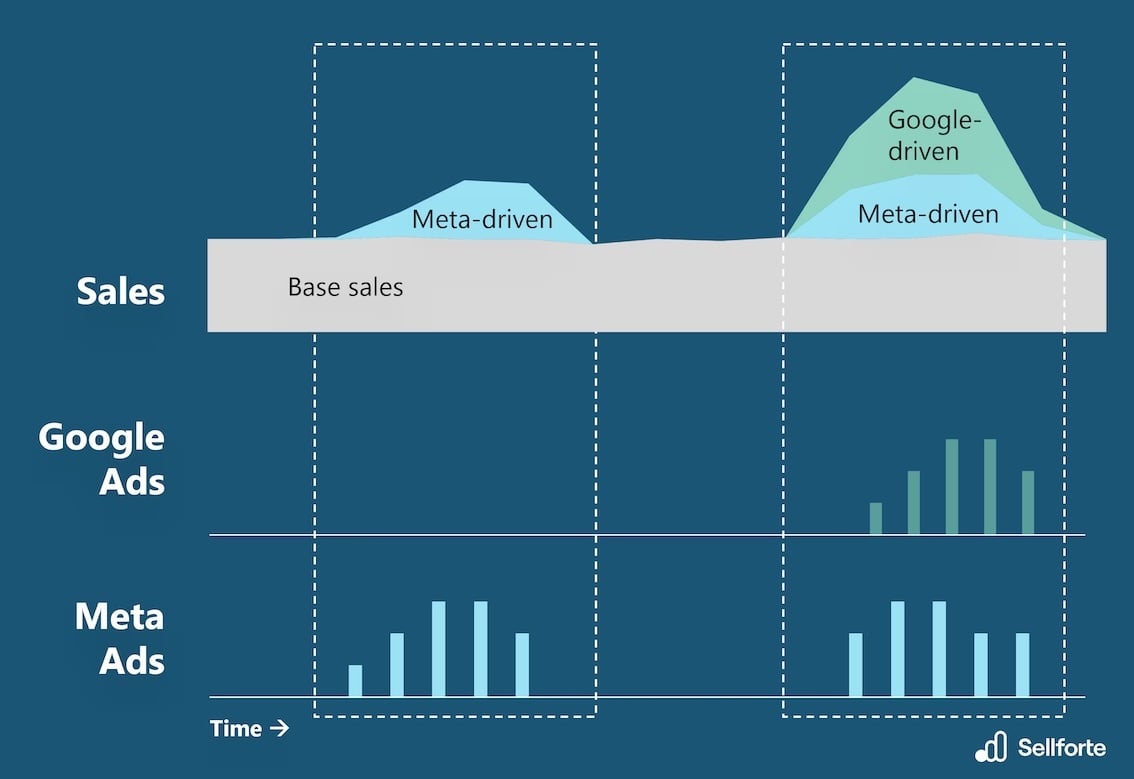
Bayesian MMM
Sellforte’s Next Generation MMM leverages Bayesian modeling, which is broadly considered as the golden standard in the industry and recommended for example by Google Research.
Bayesian modeling brings many benefits over legacy approaches and is particularly good at handling the noise inherent in MMM datasets. Bayesian models leverage “priors”, which are a method for providing the model external information on marketing’s effectiveness. Priors also enable model calibration, which is a key feature in Next Generation MMMs.
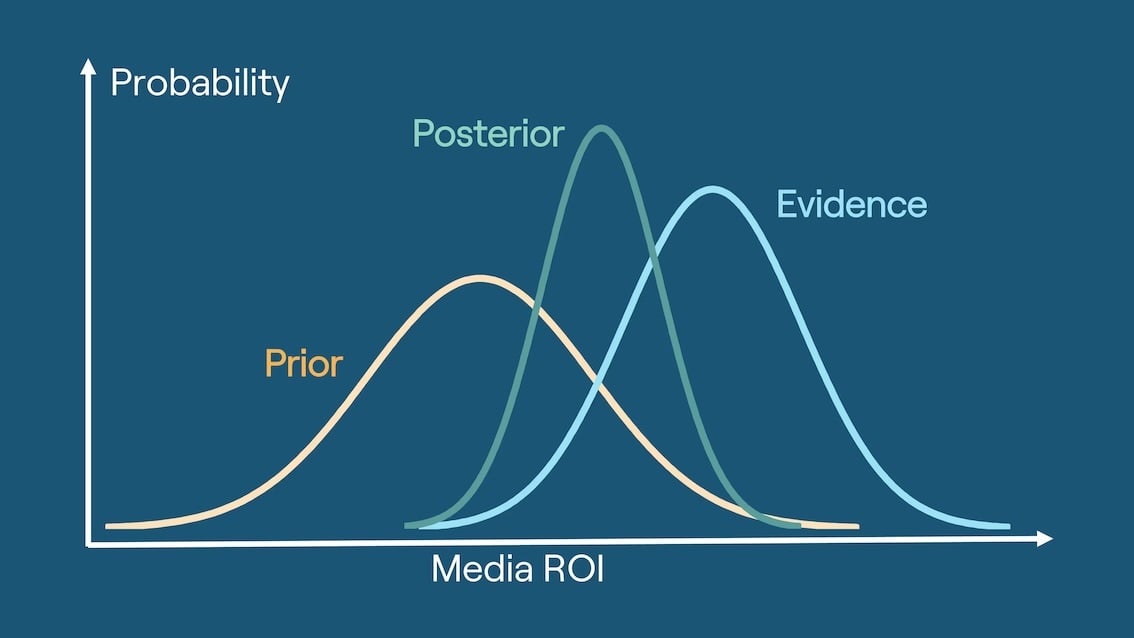
Model calibration
Sellforte’s Next Generation MMM solution leverages model calibration to deliver accurate and granular ROI estimates. Model calibration uses Bayesian models' ability to incorporate external information about marketing’s ROI in the form of priors. Using calibration data, we create informative priors, which provide the model insights on the likely range of marketing activity’s ROI.
Over the years, we have built a comprehensive calibration framework that can use two types of data:
- Incrementality tests: Conversion lift tests, Geo tests and Shutdown tests
- Attribution data: Ad platform attribution, Google Analytics 4, Multi-touch attribution
Since our calibration framework can use different types of calibration data, it works both for mature marketing teams with established incrementality testing routines, as well as for marketing teams who are yet to conduct their first incrementality test. Read more
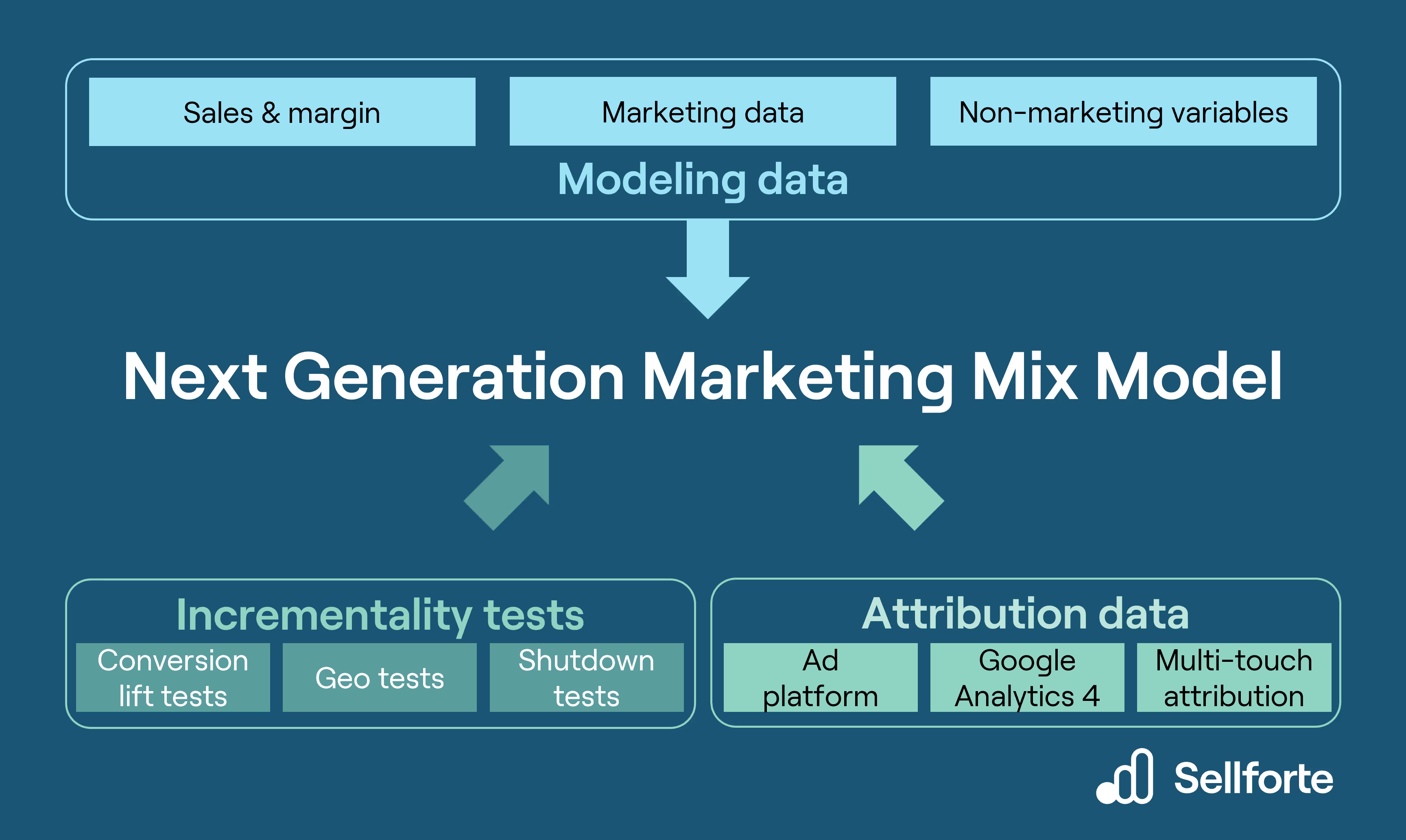
Model validation
More than half of Sellforteans are data scientists, and we put a lot of focus on ensuring high quality modeling results to our customers. Focus on quality can been seen throughout the modeling pipeline: collecting high quality data using data connectors and standard data schemas, preparing the data for modeling with advanced processing algorithms, leveraging best available modeling techniques, calibrating the model with all available ROI information, and in the end, validating the model.
We leverage several standard tests to validate the statistical performance of the model. For the users, we have built a model validation view in the Sellforte platform, which includes various validation charts, such as actual vs predicted sales, and select validation metrics, such as MAPE (Mean Absolute Percentage Error) and R2.
To help you validate the model against other performance metrics you might be used to, Sellforte's MMM dashboard includes a comparison of
- MMM-reported ROI
- Google Analytics 4 reported ROAS
- Ad platform reported ROAS
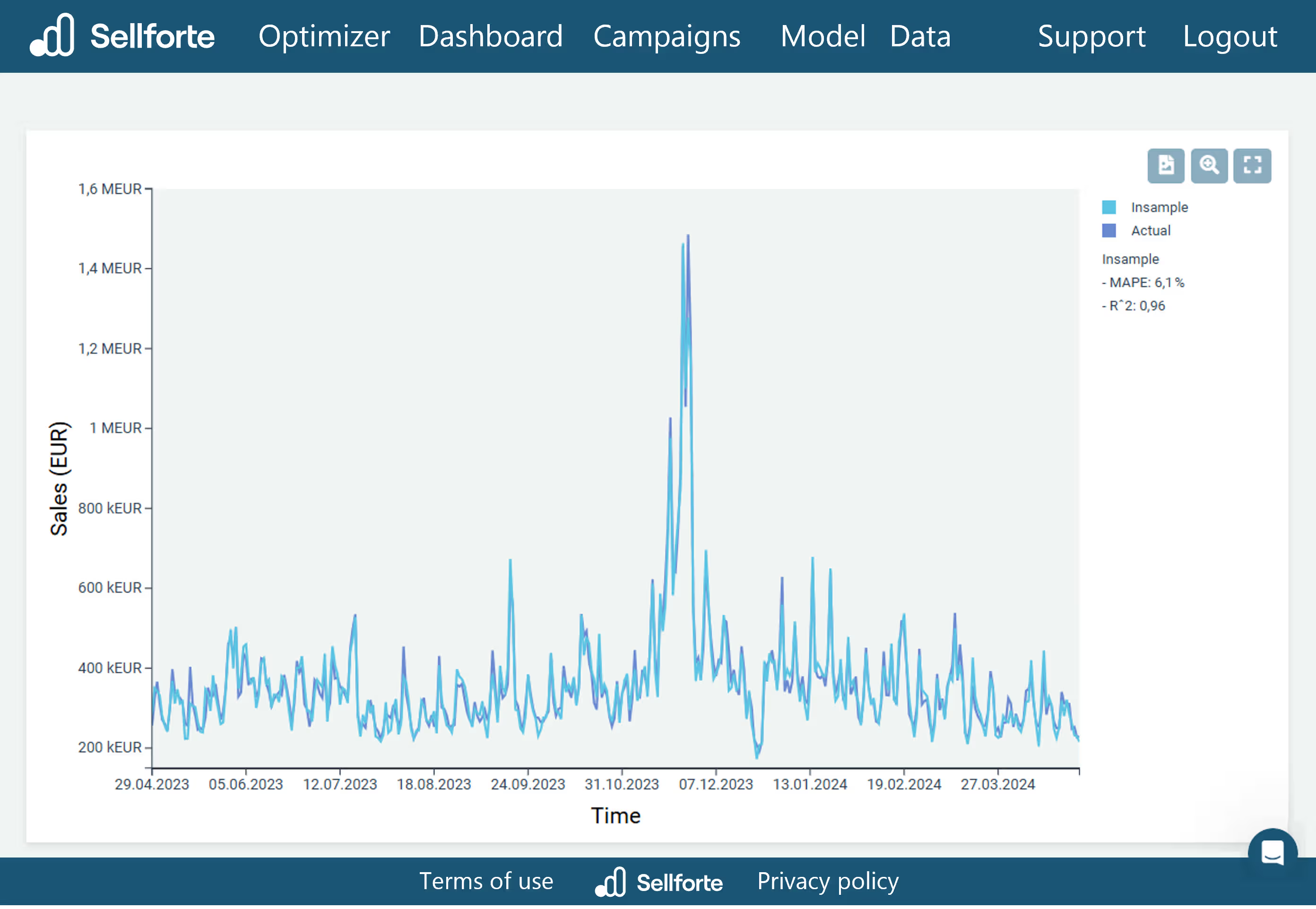