Blog
Why should you calibrate Marketing Mix Modeling results with Facebook lift tests?
December 08, 2022 | Carmen Bozga, Mikko Ervasti
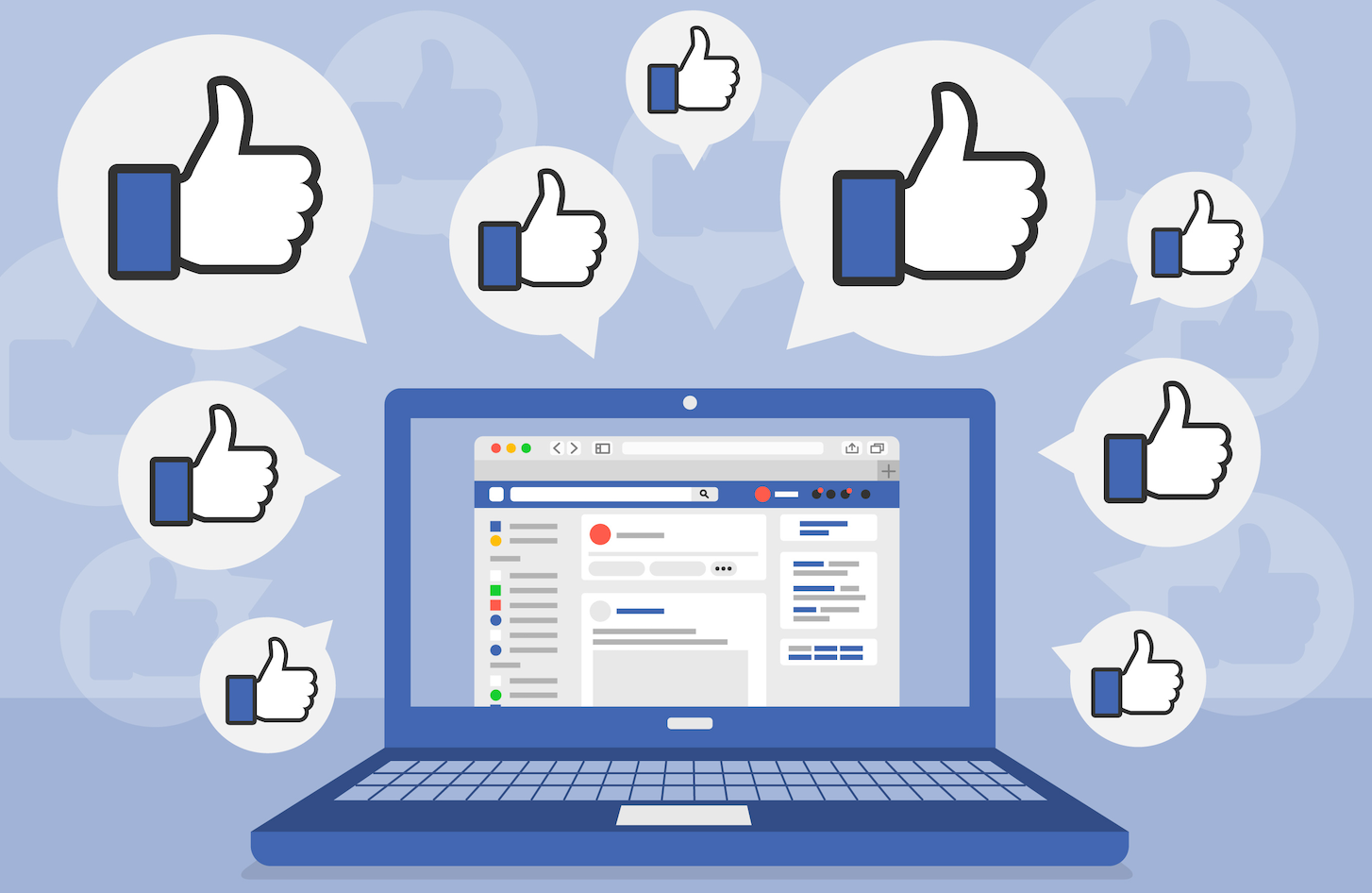
What are Facebook lift tests?
Before we talk about MMM calibration, let’s shortly go over Facebook conversion lift tests.
Lift tests are carefully conducted experiments that split your audience randomly to control and test group. By showing ads to the test group only, one can deduce the true causal incremental effect from the ads.
How does it work? Check out the steps below
- You identify your target audience
- Facebook randomly splits people between the control and test groups
- Ads are delivered to the test group only
- You share Facebook your conversion data
- Facebook compares the conversions in your test and control groups
- You get the results in your Facebook Ads Manager
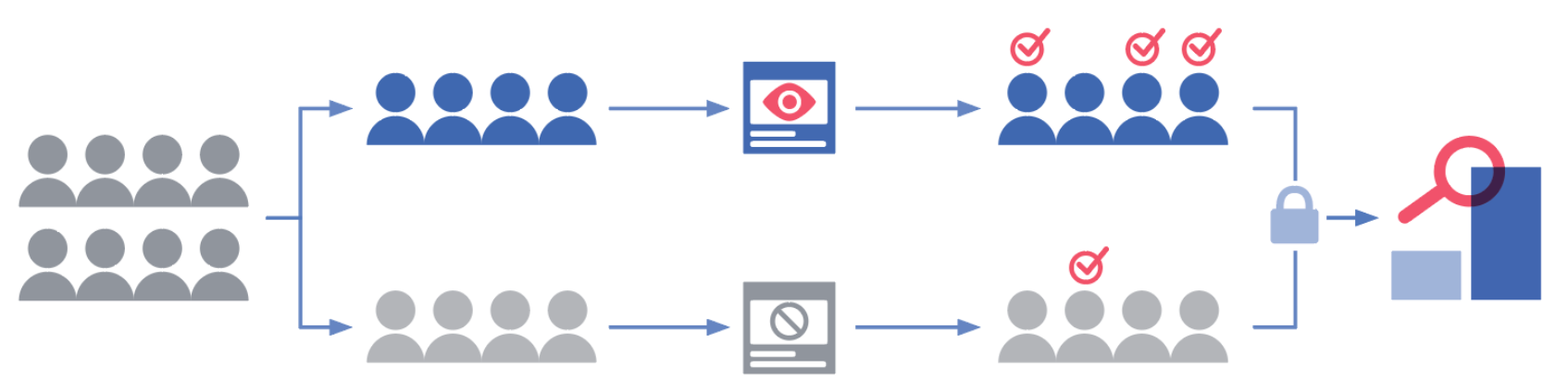
Source: For more info check out this article by Facebook
What are the benefits of Facebook lift tests?
Conducting a lift test reveals glimpses of the true performance of your ads. Typically in this scenario MMM offers much more valuable information than attribution models, because MMM tells something about incrementally. Namely the MMM shows you how many incremental conversions your ads are bringing on top of organic and other channels.
Moreover, since lift test experiments take place in a controlled setting during well-specified days, using specific campaigns etc, the ad performance can vary in time or between campaigns. For example, we noticed together with one of our clients that Facebook ads performed differently before, during and after the Covid-19 pandemic.
Are you actively thinking about global events, weather, holidays etc when you plan your ad campaigns?
What are the limitations of Facebook lift tests?
To be fully transparent, performing lift tests can be expensive. You are paying for the ads that are delivered to the test group and also the ads that are ultimately not shown to the control group. Opportunity costs aside, you might also need to plan your experiment so that there are no other large simultaneous campaigns, special promotions, other experiments or other factors that can disrupt the analysis. However, whether you perform lift test or not depends on your organization and your goals. We believe that in the long run, the cost of consistent lift tests can be seen as an investment to better understand your marketing effectiveness.
What is calibration in MMM?
The easiest way to describe MMM calibration is to say that we just tell the model what we know instead of letting the model figure out everything from the data by itself. Namely, the model can do a better job if we give it a little bit of guidance and nudge it to the right direction. Moreover, this is often business information or insights that could not necessarily be learnt from the data alone.
Calibrating the MMM model with Facebook lift tests helps finding more accurate results especially for Facebook ads, but there are also benefits to the other channels and beyond.
Now the technicalities:
MMM calibration is ideally done by matching to the exact lift tests conditions. Precisely, we let the model know the specific conversion lift obtained by the exact ads from the lift test at the exact times it was performed. The MMM model should then obtain these values (or something that is close to these values) as well as uplifts based on the lift test results. Moreover, depending on the details of the MMM model used, the model then also better understands how other similar Facebook ads perform at other times.
Quite interestingly, more accurate results for Facebook also help piece together more accurate results for other channels and baseline level as well. It is good to think MMM as a top-down method that ties multiple calibration results (such as lift test results) together into a coherent full picture. Hence the more calibration you do, the better overall MMM results you can get.
Some MMM models assume that similar channels perform in a similar fashion. Hence, if we know how Facebook behaves, we also know more how other social media ad channels behave. Essentially, if we know something more about one channel, then we can improve the accuracy of the other similar channels as well. Nevertheless, it is up to you how far you want to take this, and how far to calibrate all your media based on the available calibration results. In any case, if you want higher accuracy, you should do more lift tests for several of the ad channel platforms.
Conclusion: Sellforte calibrates your marketing mix model with your Facebook lift test results by calibrating across all historical & future modeling outputs so that you can have a more accurate overview.
What happens after the MMM model is calibrated?
Why should you calibrate Sellforte MMM with your Facebook lift test data, if lift tests already measure the true incremental uplift? Lift tests on their own do not form the full picture or help you with tactical or strategic media budgeting, gaining wider insights on your marketing, or formalizing your marketing strategy. This is where MMM comes into play. By calibrating the MMM with lift tests results, you are able to understand which campaigns are truly valuable for you, and how to optimize your marketing mix for your campaigns.
What happens if you don’t calibrate your MMM?
If there is no calibration (or regularization) of any kind, you are letting the model fully fit to the data and risk overshooting some of your parameters, such as getting too high media channel ROI values especially for your smaller investments. This is one of the typical problems in more traditional MMM approaches.
Moreover, without anchoring your results with some well-known values, such as lift test results, the results can end with high degree of uncertainty, leaving you with more questions than answers. However, when you start adding calibration data and assumptions, it becomes easier for the model to start converging to the real media effectiveness of other channels as well, finally revealing the picture how your marketing is performing.
Calibrating media also helps to find more accurate baseline levels, i.e. the organic share of conversions. This is one of the benefits of MMM in comparison to attribution or other measuring techniques. It’s not just finding better media channel uplifts but also the base.
Let`s recap:
- A lift test shows the incremental impact a specific ad has on a channel.
- Calibrating the Facebook lift test results into your Sellforte MMM gives you more accurate all around results across your other ad platforms & especially on Facebook
- You get more accurate ROI predictions for your ad platforms
- You can make better marketing investment decisions in the future
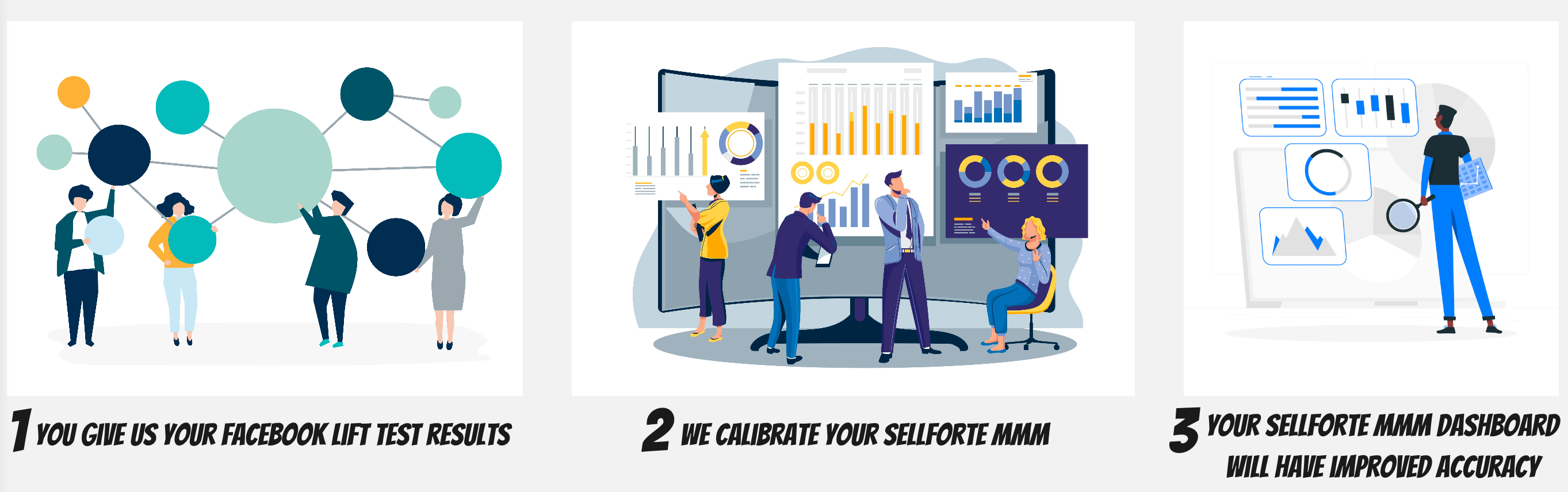
We hope that this article was able to offer you more clarity in your future choices! If you are curious about Sellforte, you can try out our MMM free here!
We also recommend this blog posts: Why should I use Marketing Mix Modelling when I have Google Analytics
Curious to learn more? Book a demo.
Related articles
Read more postsNo items found!