Blog
How accurate are Marketing Mix Modeling results typically?
February 17, 2023 | Carmen Bozga, Chris Kervinen, Paavo Niskala
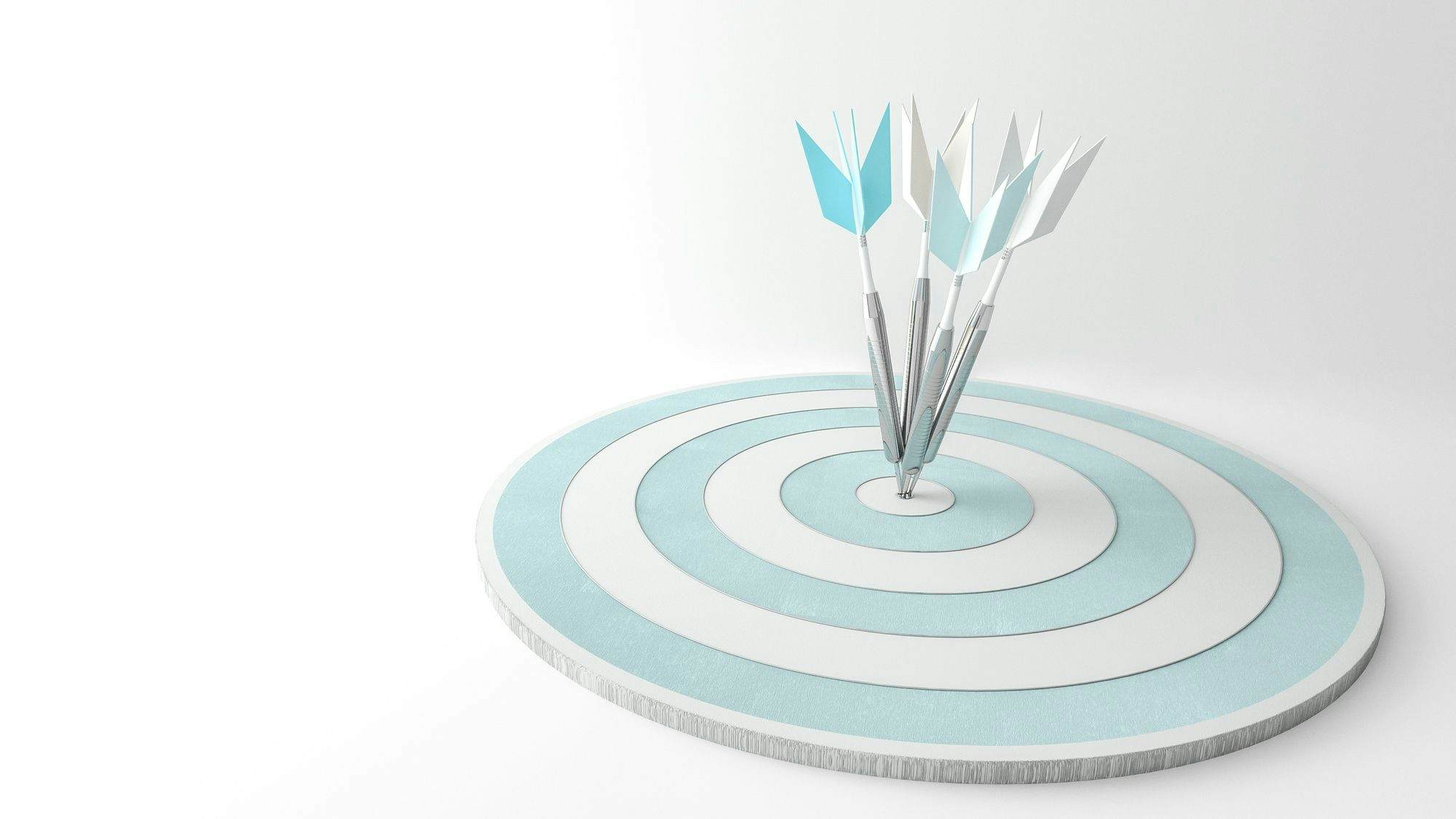
We understand that businesses are always looking for ways to improve their marketing strategies and measure their results. Marketing mix modeling is an incredibly useful tool that can help marketers achieve their goals, however, it's important to understand how reliable and accurate the results that MMM provides can be. One common question that pops up is the level of accuracy of the results obtained from such models. The accuracy of a model depends on several factors such as: the quality of data used to build it, as well as the assumptions and parameters utilized. In this blog post, we will dive into the accuracy of marketing mix modeling results, examining the different factors that can influence the model's accuracy and the level of confidence we can have in its predictions. Whether you are considering investing in marketing mix modeling for your business or simply seeking to understand the accuracy of its results, we have you covered in this blog post!
Before diving into this blog post, let’s clarify two crucial terms for this topic: probability and accuracy.
Accuracy is a measure of how close something is to being correct. Imagine you are playing a game of darts, and you are trying to hit a bullseye, which is the center of the target. The closer your darts land to the bullseye, the more accurate you are.
Probability is a way to talk about the chances of something happening. For example, when you flip a coin, there is a 50% chance that it will land heads and a 50% chance that it will land tails. These chances can help us make predictions about what might happen in the future.
Think of probability like a guess, or a prediction, of what might happen. The more information we have, the better our guess can be. And, just like with accuracy, the closer our guess is to what actually happens, the better it is! The two concepts are interlinked, however, detrimental different in terms of Marketing Mix Modeling (MMM) solutions.
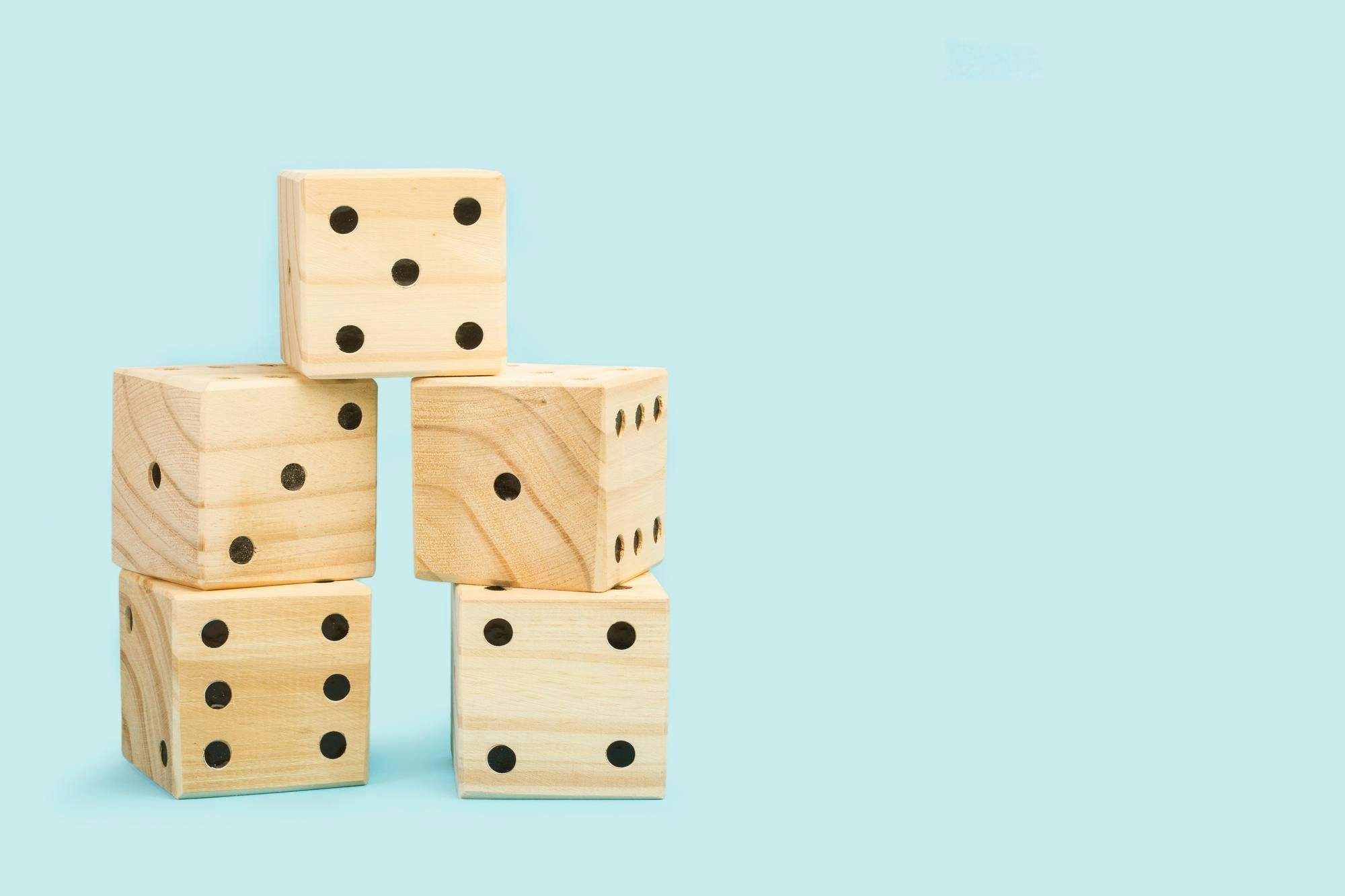
Deterministic or probabilistic?
It's important to understand the differences between deterministic and probabilistic marketing mix modeling (MMM).
- Deterministic MMM solutions are based on the assumption that there is a direct cause-and-effect relationship between marketing activities and business outcomes, while probabilistic MMM recognize that the relationship between marketing activities and business outcomes can vary due to factors beyond the marketer's control.
- Probabilistic MMM uses various advanced techniques such as regression analysis, time-series analysis, and Bayesian methods to capture the randomness and variability of data, resulting in more accurate predictions. These models use statistical methods to estimate the probabilistic relationship between marketing activities and business outcomes, incorporating a measure of uncertainty and variability in the model's predictions.
- Deterministic MMM uses historical data to determine the impact of specific marketing activities and assumes that the relationship between these activities and outcomes is constant over time. In other words, the model assumes that if you change a particular marketing activity, you can predict the exact change in business outcomes that will result.
- Deterministic models are simpler to develop and implement, but may not be as accurate, whereas probabilistic models provide a more realistic understanding of the complex relationship between marketing activities and business outcomes – although they may require more data, time, and expertise to develop and interpret.
Simply put, the main difference between deterministic and probabilistic MMM is the degree of complexity and accuracy of the model. Bayesian models are inherently more accurate than deterministic models.
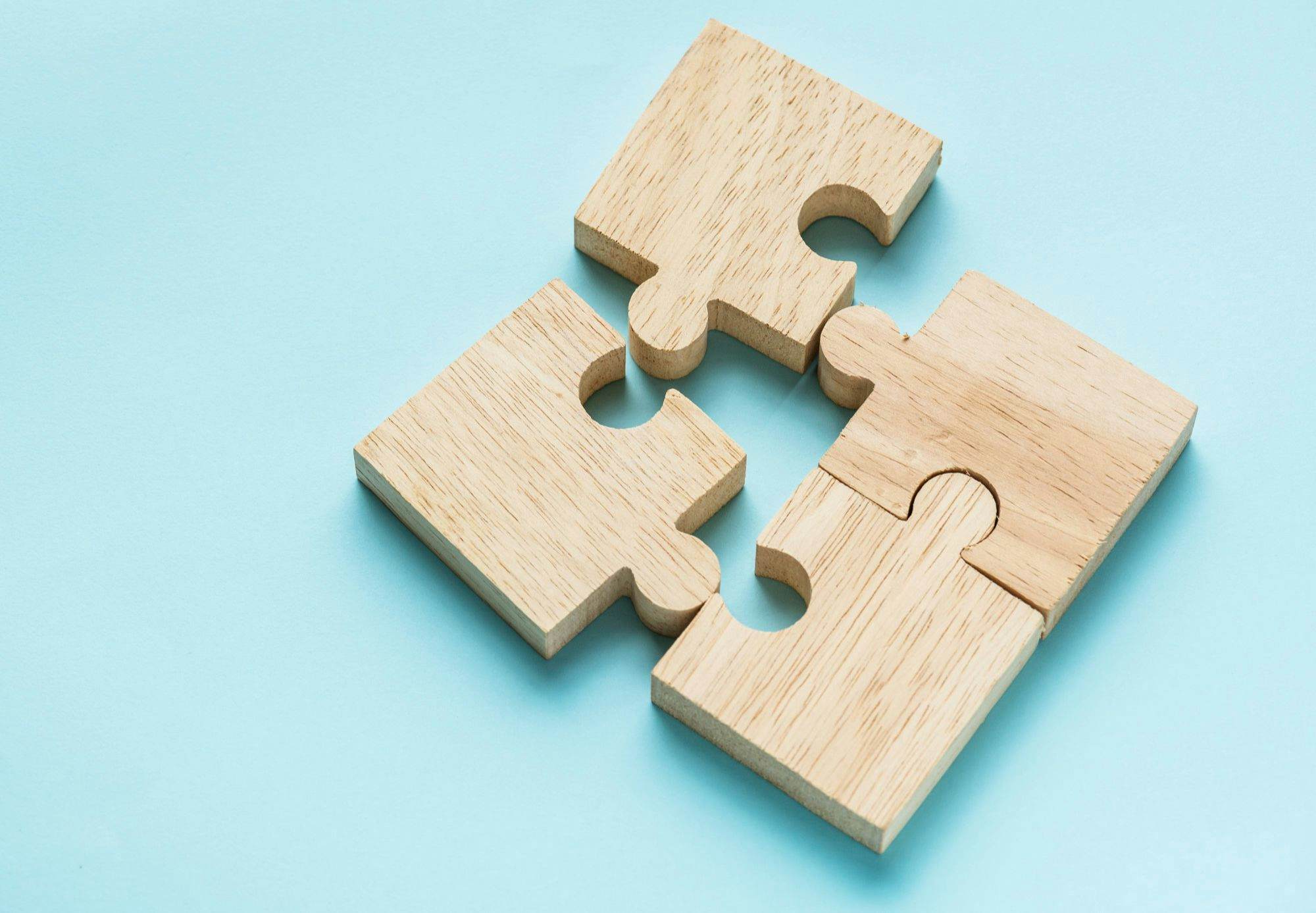
What are Probabilistic Bayesian models and how do they compare to other marketing measurement solutions such as Multi Touch Attribution?
Probabilistic Bayesian marketing mix modeling (BMMM) has gained popularity in recent years due to its flexibility and ability to provide probabilistic estimates of the model parameters and predictions. In contrast to deterministic MMM, BMMM incorporates probabilistic elements into the model, which allows for more accurate and precise estimates of the impact of marketing inputs on sales.
BMMM uses Bayesian statistical methods to calculate the posterior distribution of the model parameters. This approach considers the observed data and prior knowledge, leading to more accurate estimates of the model parameters. The posterior distribution also allows for uncertainty and randomness in the data.
With BMMM, you can take advantage of the non-linearity and interactions between marketing inputs and sales outcomes, resulting in more precise and reliable estimates of each input's effect. What's more, you can also factor in your prior knowledge or beliefs about the parameters, which can help boost accuracy - especially when you're working with less data.
To put it simply, BMMM is like putting together a big puzzle. You have some pieces of the puzzle, like how much money a company spends on advertising and how many things people buy. But there are some missing pieces that you don't know yet.
With BMMM, you use math to fill in the missing pieces of the puzzle. You look at the pieces you already have, and you use them to make a good guess about what the missing pieces might be. Then you check your guess against the actual results, and you use what you've learned to make even better guesses in the future.
This method helps you make more accurate guesses about how different things affect what people buy. It also helps you factor in any uncertainties or randomness that might be happening. And you can even use what you already know about the puzzle to help you make better guesses. Moreover, it is the opposite of a black box model. There is complete transparency regarding its functioning principles.
How does Bayesian MMM compare to Multi Touch Attribution?
Multi-Touch Attribution (MTA) is another popular approach to measure the impact of different marketing channels on sales outcomes. MTA aims to allocate the credit for a sale to each marketing touchpoint that contributed to the conversion, based on some predetermined rules or models. However, MTA is often criticized for its over-reliance on ad hoc heuristics and assumptions, which can lead to biased and unreliable estimates of the contribution of each touchpoint.
While multi-touch attribution has its advantages, it is often criticized for its ad hoc assumptions and lack of rigorous statistical modeling. Compared to MTA, BMMM provides a more rigorous approach to measuring the impact of marketing inputs on sales. BMMM is based on a well-defined statistical model that incorporates prior knowledge and observed data to estimate the posterior distribution of the model parameters, which allows for more accurate and precise estimates of the effects of each input. Moreover, BMMM can handle various types of data, including time series, panel data, and aggregate data, which makes it suitable for a wide range of marketing applications.
You can find an in-depth comparison of MMM and MTA here.
How is the accuracy of MMM results measured?
Now that we have discussed the differences between deterministic and probabilistic approaches to MMM, let's move on to the accuracy of MMM results. How accurate are the results of MMM models on different levels? How can we validate the results? What are the factors that can improve results accuracy? These are all important questions to consider when evaluating the accuracy of MMM results.
Measuring the accuracy of MMM can be challenging because ultimately you should not be interested in how well the MMM predicts e.g. the total sales of your business but what kind of uplifts it predicts for a variety of marketing activities. If you look at TV as a media channel, it is pretty much impossible to predict or know exactly how much incremental sales it drives. Since the true incremental sales impact of each individual marketing input is not known, it can be difficult to determine the exact accuracy of the model's estimates.
However, there are ways to measure the accuracy of the MMM models by using MAPE or R2 metrics.
- MAPE stands for Mean Absolute Percentage Error. It measures how far off the model's predicted values are from the actual values, as a percentage of the actual value. For example, if the model predicted 100 sales and the actual number of sales was 110, the error is 10%, because the model was off by 10 sales. MAPE calculates the average error as a percentage of the actual value, so it tells us how accurate the model is, on average.
- R2, on the other hand, stands for the coefficient of determination. It measures how well the model's predicted values match the actual values. R2 ranges from 0 to 1, with 1 meaning a perfect fit and 0 meaning no fit at all. R2 takes into account the variation in the actual values and how much of that variation is explained by the model's predicted values. A higher R2 value means the model is a better fit for the data.
In simpler terms, MAPE tells us how often the model's predictions are off by a certain percentage, and R2 tells us how well the model fits the actual data.
MAPE and R2 metrics are important measures of the accuracy of Marketing Mix Models, but they have their limitations. These metrics can tell us how well the model is able to predict sales, but they don't necessarily tell us how well the model is able to capture the impact of specific marketing activities, such as advertising or promotions. Therefore, the impact of marketing activities can be limited compared to other factors that affect sales, such as seasonality or pricing. In addition, there are often many different media channels and campaigns to consider, which can be difficult to accurately represent as independent variables in the model.
This means that even if the model has a high predictive power, it may not be able to accurately capture the true impact of individual marketing activities.
An additional way to improve the accuracy of MMM is through lift tests. You can read more about it here.
However, lift tests alone have their limitations. There is always some level of uncertainty in the results, and the effectiveness of a media channel can vary over time due to various factors such as changes in user behavior or changes in the way user data is tracked. Additionally, the results of lift tests may be influenced by other factors besides the media channel, such as external events or changes in the competitive landscape. However, using lift tests together with MMM prove to be rather effective at calibrating the model.
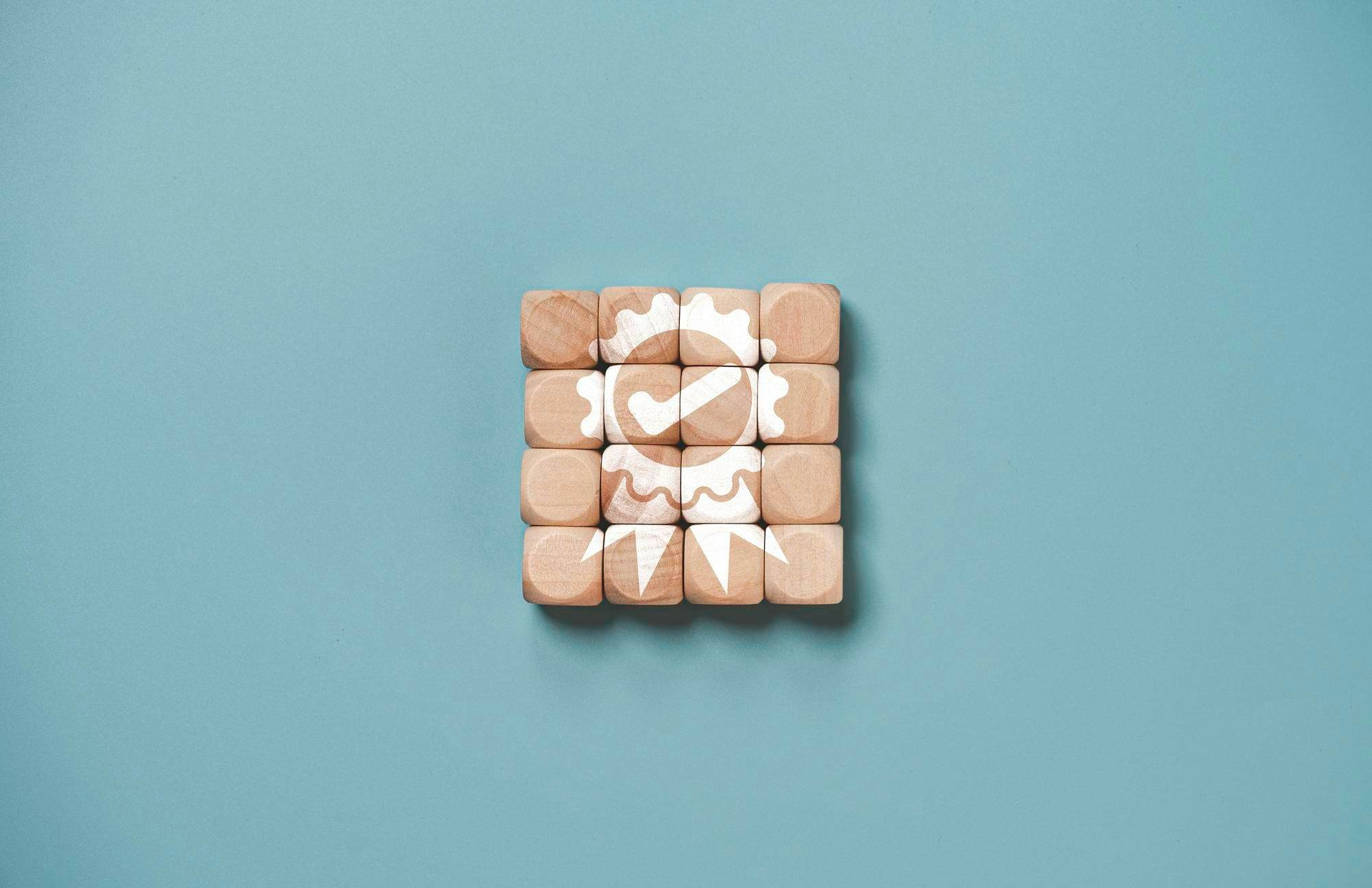
How accurate is MMM in practice?
The accuracy of MMM results can vary in practice depending on a several of factors, however, it's generally estimated that MMM can achieve an accuracy of 80-95% for predicting the impact of marketing on sales, depending on the granularity of the results you want to interpret. This range is based on industry standards and best practices, and it can vary depending on the quality and quantity of the data used, the complexity of the model, and the modeling techniques employed.
Despite its best efforts, MMM cannot guarantee 100% accuracy in its predictions due to the uncertainty that comes with statistical models. The model's accuracy is only as good as the data and assumptions that go into it. To reduce this uncertainty, it is important to consider external factors such as market conditions, competition and customer preferences before making decisions based on the model's predictions.
If we were to compare MMM with MTA in terms of accuracy, then it would be something along the lines of comparing apples and oranges since they approach the problem of measuring the impact of marketing activities in different ways. MTA can guarantee 100% accuracy since it attributes every touchpoint to a media channel.
However, it's important to remember that the difference in accuracy between MTA and MMM is not the only factor to think about when looking at these approaches. MMM is often seen as more realistic and reliable because it takes into account the complex interactions between marketing channels and the external environment, such as changes in consumer behavior and market trends. On the other hand, MTA assumes a fixed formula for all transactions, which can lead to inaccurate or incomplete results in the event of a market shift. Additionally, MMM might be better for longer-term planning and budget allocation, considering it takes into account the full impact of external factors and provides a more comprehensive view of the expected financial results. Alternatively, MTA is more suitable for short-term optimizations since it is a simpler approach that focuses on immediate results without considering potential long-term effects.
Marketing, or reality in general cannot be modeled 100%, and it's important to understand the limitations and potential risks associated with relying on any modeling approach, including both MTA and MMM. While MTA may provide attribution results that appear to be more accurate due to its methodology of assigning 100% credit to each touchpoint, it may not necessarily reflect the true impact of marketing activities on consumer behavior.
MMM takes a more holistic approach to understanding the impact of marketing on sales, taking into account the complex interactions between various marketing channels and external factors such as seasonality, economic conditions, and competitor activity. As a result, MMM is generally considered to be a more realistic and reliable approach, even if it cannot achieve 100% accuracy.
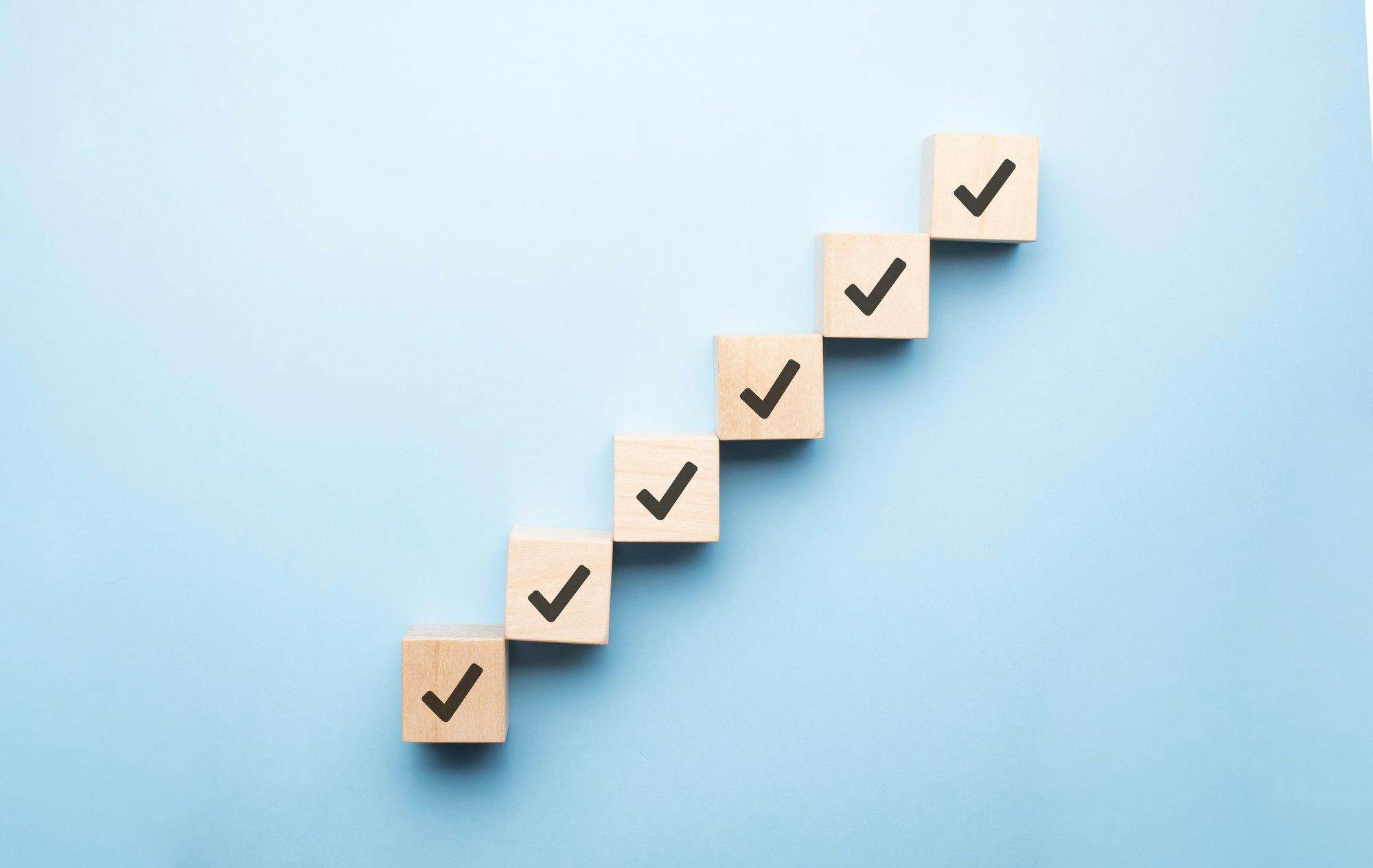
How can we validate the results?
As mentioned above, Bayesian MMM results can be constantly measured and adjusted which allows them to be as close as possible to the actual reality. However, let’s have a structured overview of what we discussed so far.
In order to ensure the accuracy and reliability of Marketing Mix Models (MMM), it's important to validate the results using a variety of techniques. These techniques can be broadly categorized into three types of validation: technical validation, performance validation, and business validation.
- Technical validation involves using Bayesian statistical inference to ensure that the models are performing properly. This approach uses metrics that are automatically checked every time the model is trained on data, which helps to ensure that the models are consistently performing at a high level.
- Performance validation allows for standard accuracy metrics such as MAPE and R2 to evaluate the model's performance. These metrics are calculated automatically by the modeling pipeline and provide a quantitative measure of the model's accuracy.
- Business validation means applying standard business validation methods to verify that the outputs of the MMM models make sense from a business perspective. We do this by having our Customer Success Managers and Data Scientists to review the results and ensure that they align with the business goals and objectives of the organization.
In addition, it's also possible for customers to provide ground truths, such as lift tests for Meta's platforms, which the MMM models can be validated against. This allows the models to be benchmarked against real-world data, which can help to further validate their accuracy and reliability.
By using a combination of technical, performance, and business validation techniques, businesses can gain greater confidence in the results produced by their MMM models, and make more informed decisions about how to allocate their marketing budgets.
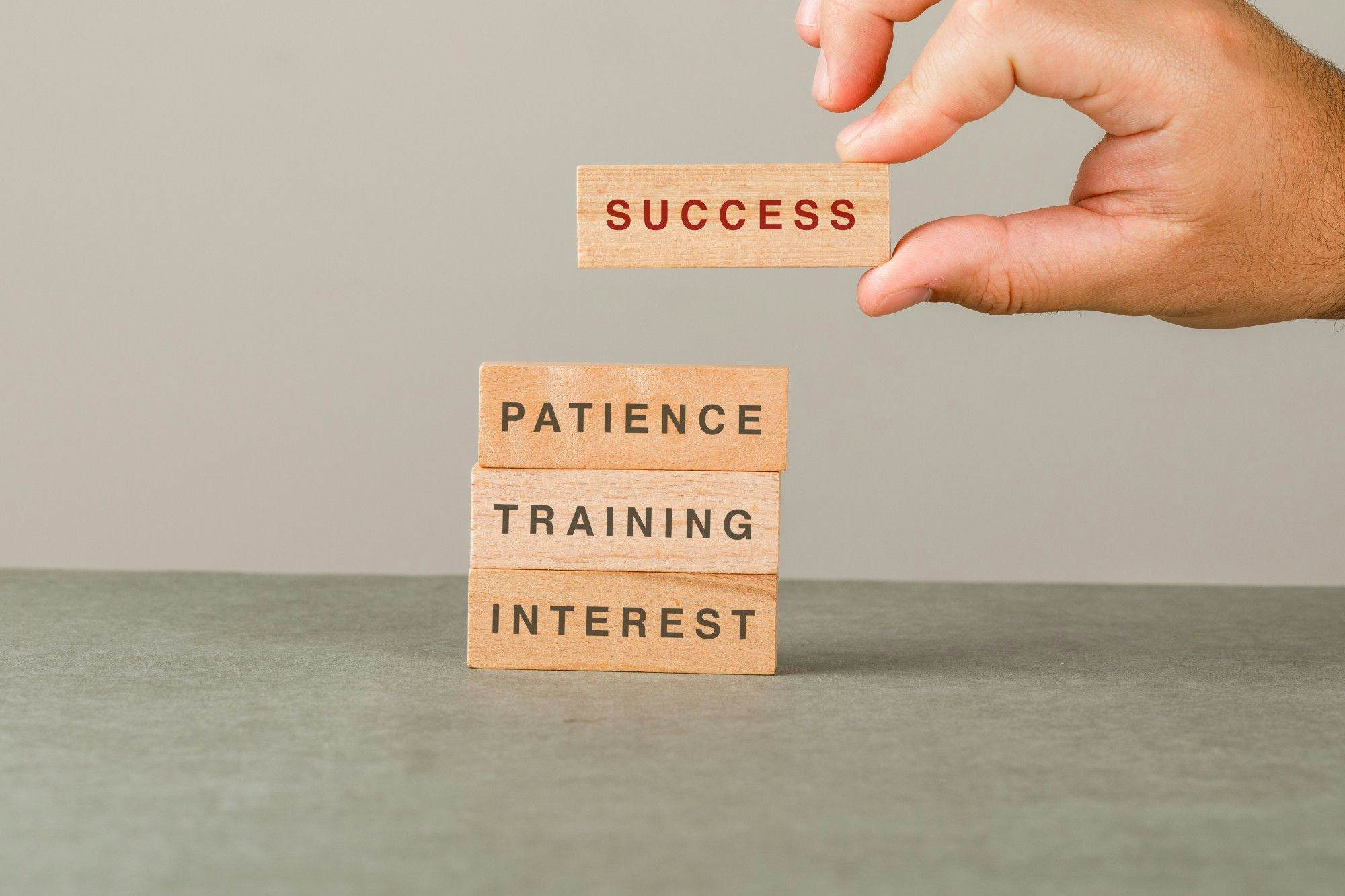
What are the factors that can improve results accuracy?
Aside from what we mentioned already, there are a few other factors that can contribute to the accuracy of the MMM model.
- Data quality: High-quality data is essential for accurate MMM modeling. This includes sales data, marketing spend, and other relevant variables.
- Granularity of data: The more granular the data, the more accurate the results are likely to be. This means having data at a more detailed level, such as by product, region, or channel.
- Proper attribution of marketing spend: Properly attributing marketing spend to specific channels or campaigns can help to more accurately determine their impact on sales outcomes.
- Incorporation of external factors: seasonality, events such as the pandemic, and economic indicators can help to more accurately model the impact of marketing activities.
- Robust statistical methods: for example Bayesian modeling can help to account for uncertainty and variability in the data, leading to more accurate results.
- Ongoing validation and optimization: Regularly validating and optimizing MMM models can help to ensure that they remain accurate and relevant over time, as the business and marketing environment changes.
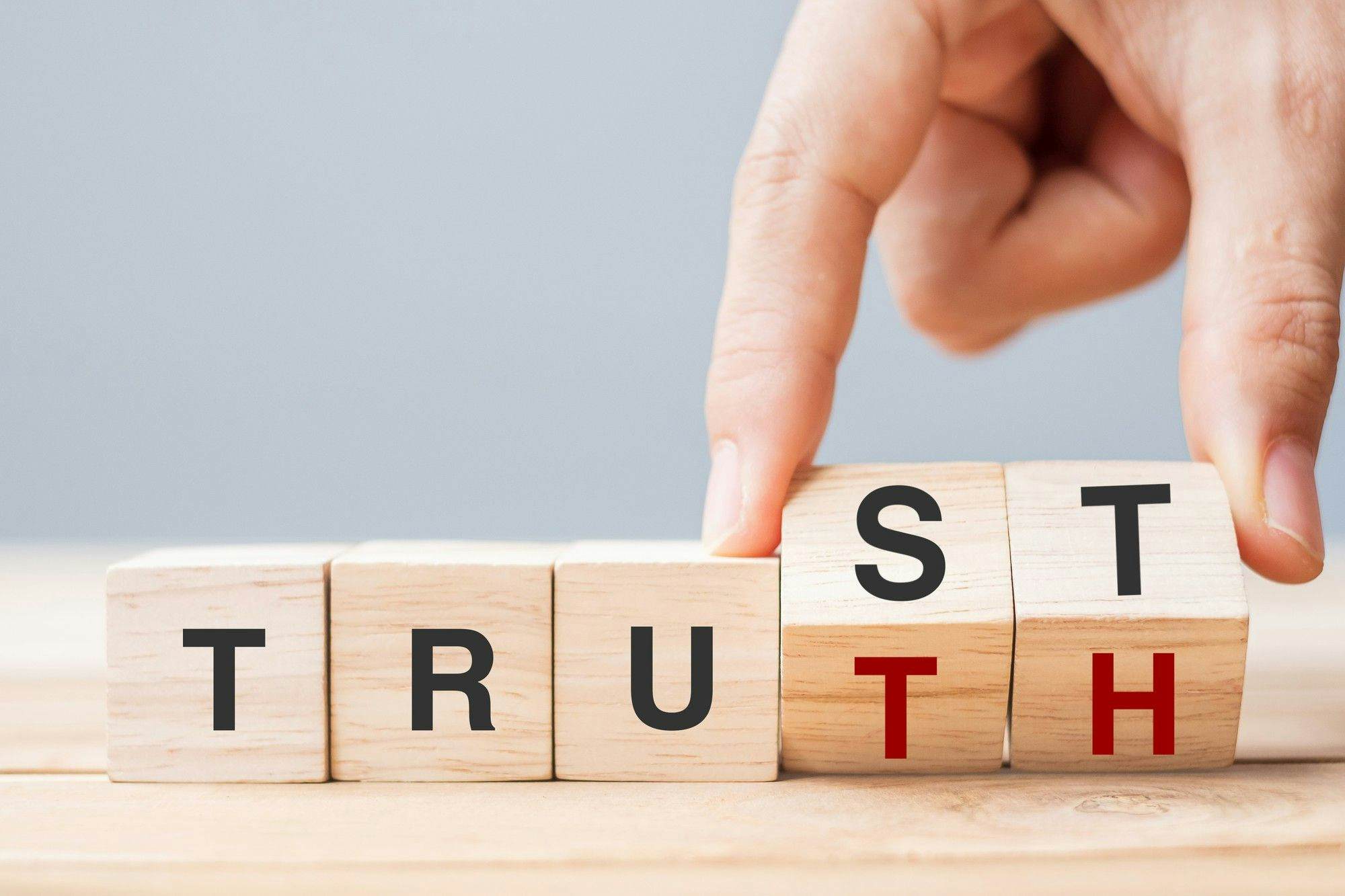
Can you trust MMM results?
Don't worry, businesses can trust that the results of their Marketing Mix Models (MMM) are totally on point! But, there's always room for improvement with MMM.
We highly recommend taking the validation and optimization of MMM models seriously, using a variety of technical, performance, and business validation methods. This will help marketers to make more informed decisions about their marketing activities, enabling them to reach their business objectives in a more effective way.
By using the best possible data, improving attribution methods, and accounting for external factors, businesses can continue to tweak their MMM models to get even more accurate and useful insights.
Moreover, it is important to recognize that the marketing landscape is constantly evolving. Therefore, marketers must stay up to date with the latest trends and technologies in marketing, and we work closely with our clients to incorporate their feedback and insights into our modeling processes. And as marketing trends and technologies change, MMM models can evolve and improve to stay relevant. With ongoing calibration and validation, businesses can feel totally confident in their MMM models and the insights they give.
Curious to learn more? Book a demo.
Related articles
Read more postsNo items found!