Methodology
A glimpse under the hood of Sellforte's powerful Marketing Mix Modelling platform
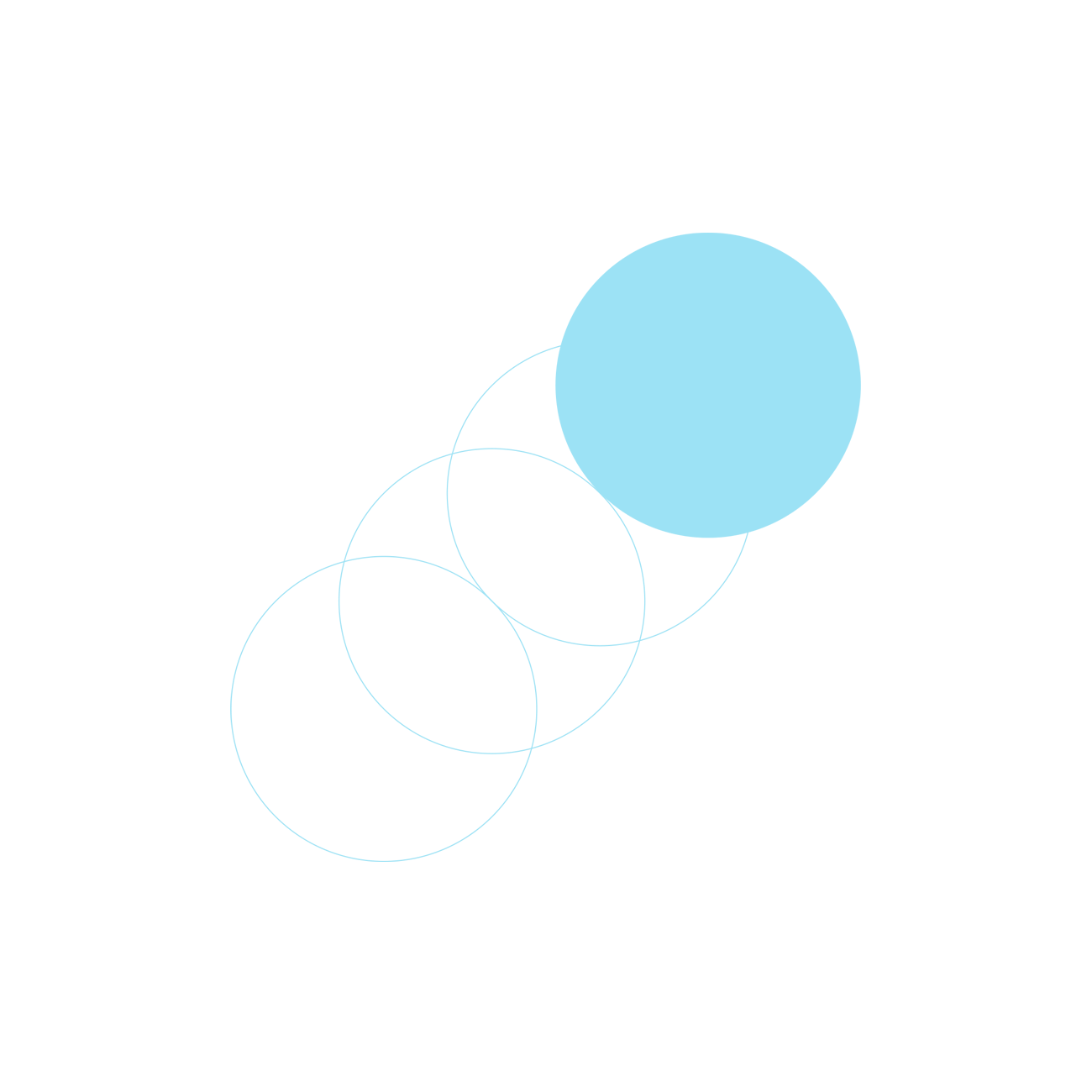
Overview
Sellforte's analytics toolbox contains a variety of methods, but we are especially big believers in Bayesian inference. It lies in the core of many of our product features, including our Marketing Mix Models.
Hierarchical Bayesian inference enables deriving robust and actionable insights with little data. Although Big Data often steals the headlines, Small Data is typically more challenging. It’s the kind of data that emerges when the ambition and granularity of the analytics increases.
The robustness of Bayesian inference results from putting together prior beliefs and evidence from the data. Domain expertise plays a key role also when building the models component by component, choosing which datasets to include, and utilizing the hierarchical structures in the data. This way the model operates within a well-defined business context and not as a blank slate.
Additionally, Bayesian inference allows building models that are diagnosable and explainable – you don’t have to put your faith in a black box.
Bayesian hierarchical modelling
Sellforte’s approach on Marketing Mix Modelling (MMM) is Bayesian hierarchical modelling methodology which has been the gold standard for MMM since 2017 when Google’s research team decided to sum up the problems with current methodologies in MMM.
Bayesian hierarchical models enables usage of priors in modelling which helps to manage the noise of the data, but also the model to learn from data when there are statistically significant differences e.g. TV channel Y has higher ROI than TV channel X.
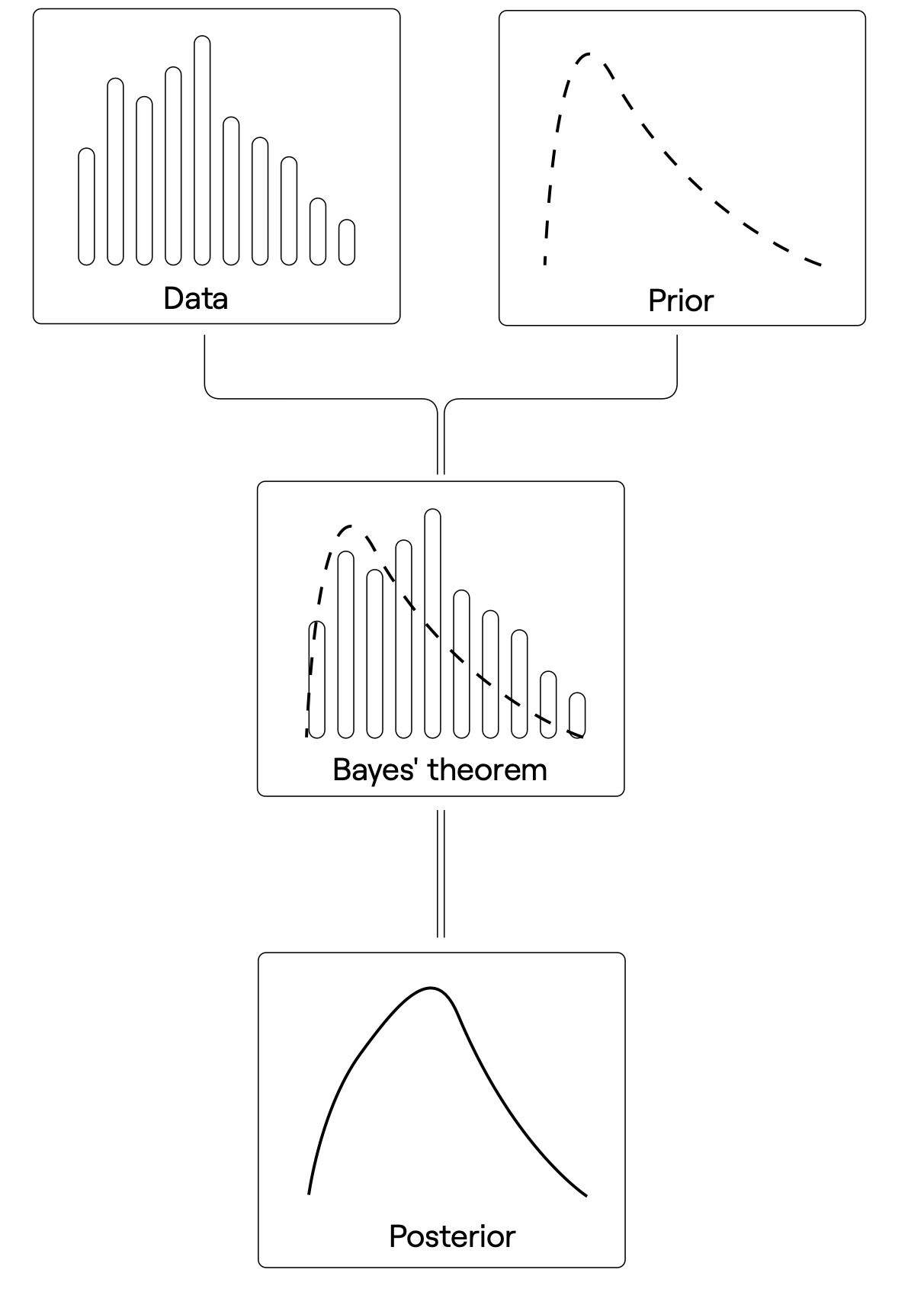
Why Bayesian methods?
1. Combines evidence from data and business context via priors
Assumptions are made more explicit and allows getting sensible results with small data, regularizing the models: it really is the perfect match for marketing mix modelling.
2. Hierarchical models leverage structure in the data
Makes models more complex but regularizes the model even further.
3. Models output probability distributions of parameters and outcomes
Uncertainty is systematically and inherently incorporated into the models, enabling the evaluation of confidence in the outcomes.
4. Systematic way of updating the model with new data
Results of the previous model become priors of the new model.
5. Mature and actively developed tech and tools to support a rigorous modelling workflow
The performance of the models improves and can be evaluated systematically: it’s not a black box.
Single timeseries vs. Multi timeseries
Single timeseries
Fit e.g. each category – sales channel combination independently, but the media features/parameters are hierarchical.
E.g. single TV channel might have sparse data, this helps control the model not to predict crazy results for this channel and keeps TV “coherent”.
Multi timeseries
Fit multiple “sales groups” (e.g. all the categories within a sales channel) simultaneously.
Hierarchies exist within e.g. the same media group but also for the same media in e.g. different product groups.
Helps solve “why is TV good for cat food but bad for dog food” type of issues.
Everything starts from the base
As in Marketing Mix Modelling it’s important to find right baseline, otherwise all modelling results will be off, Sellforte uses various of methods with external data sets to set up base line properly:
Always included:
- Yearly and weekly seasonalities
- Standard holidays for countries in scope
Provided by Sellforte depending on the use case:
- COVID-19 Effect
- Weather
- Consumer confidence
Provided by the Customer depending on the use case:
- Competitor activity
- Number or stores / distribution
- Organic traffic
Long-term effects
One of the hardest problems in marketing effectiveness is to define monetary value for long-term effects in brand-building campaigns. The problem sure doesn’t get any easier since the marketer's data is not typically supporting this since it needs to be extremely well littered and from a long period of time to get to the results.
If data sets are in shape one can use Sellforte’s proprietary Long-Term Effects framework (LTE) which can be implemented to break baseline sales to include monetary value for the long-term effects of brand building. This part of the sales can be called Brand Equity which is more known amongst marketers.
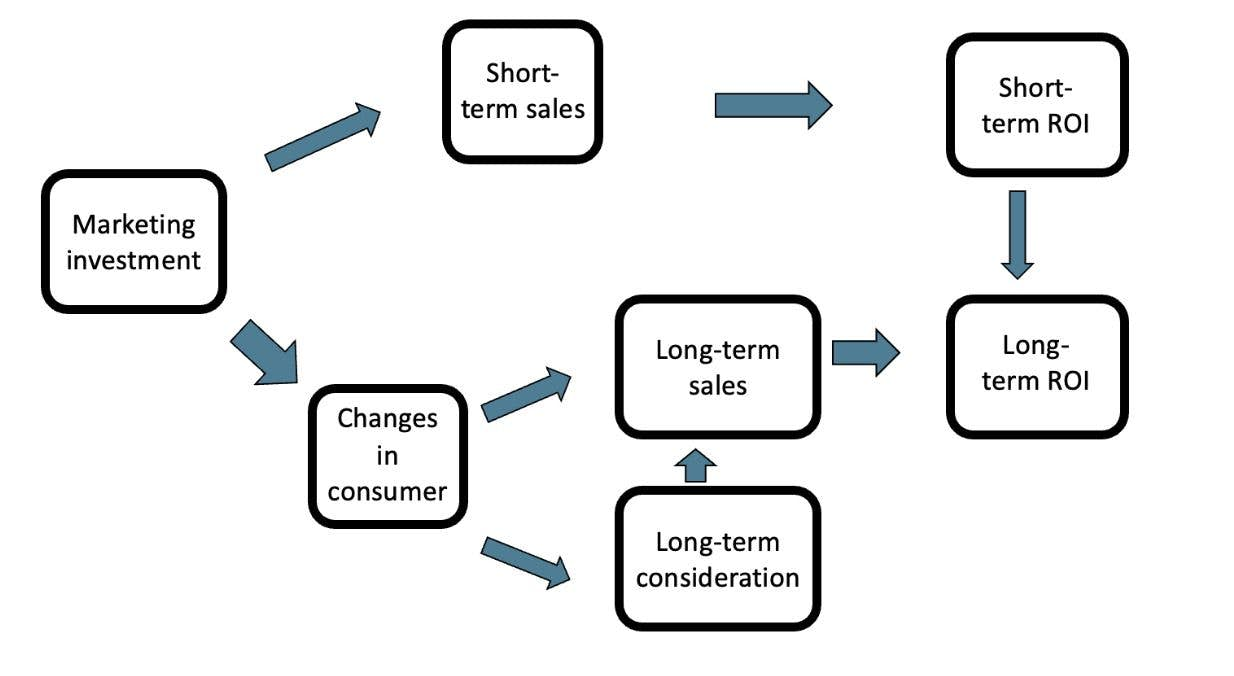